Value of Intraoperative Data Unclear in Mortality Risk Prediction Models
Researchers have found that adding continuous intraoperative data to information used by machine learning-based mortality risk prediction models may not improve predictions.
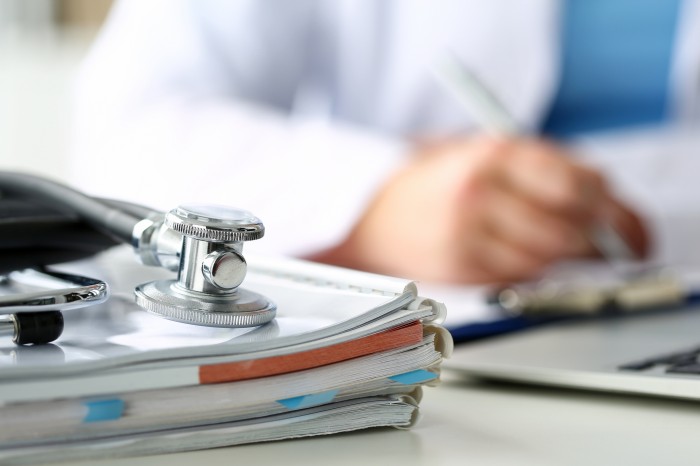
Source: Getty Images
- A new study published in JAMA Network Open found that adding continuous intraoperative data to routinely collected perioperative data used by machine learning (ML)-based mortality prediction models following cardiac surgery did not improve the models’ predictions, indicating that the value in adding these data to future models is unclear.
According to the study, postoperative mortality risk in cardiac care is associated with several preoperative, intraoperative, and postoperative factors, including age, comorbidities, and decreased preoperative kidney function. Current risk stratification methods leverage these factors to predict short- and long-term risk, but the value of using routinely collected, more complex intraoperative and postoperative data for these predictions is understudied.
The study authors further noted that ML-based prediction models have been developed in this area. Some models predict complications and mortality more accurately than traditional risk-scoring methods. ML models also have the capacity to process and use large amounts of data, such as the continuous data generated before, during, and after surgery.
Because of this, the researchers set out to compare ML-based prediction models using preoperative, intraoperative, and postoperative data to predict short- and long-term mortality after cardiac bypass and valve operations. They began by gathering data from 9,415 adult patients undergoing a first-time valve operation, coronary artery bypass grafting, or a combination of both between 1997 and 2017 at the University Medical Centre Groningen in the Netherlands. Mortality data for study participants were obtained in November 2017.
The researchers developed prediction models to forecast postoperative mortality rates at 30 days, one year and five years. To assess model discrimination and performance, the research team relied on area under the receiver operating characteristic curve (AUROC) measurements. To investigate the contribution of preoperative, intraoperative hemodynamic and temperature, and postoperative factors to patient mortality, the researchers used Shapley additive explanations (SHAP) values.
Overall, mortality rates for the study cohort at 30 days, one year, and five years were 2.8 percent, 4.5 percent, and 6.5 percent, respectively.
Models including perioperative data — that is, preoperative, intraoperative, and postoperative data — achieved AUROC values of 0.82, 0.81, and 0.80 for 30-day, one-year, and five-year mortality rate predictions. Models using only postoperative data achieved similar performance, with AUROC values of 0.78 for 30-day, 0.79 for one-year, and 0.77 for five-year mortality rate predictions.
Further, the authors found that models based on all perioperative data provided less clinically usable predictions with lower detection rates. They also found that postoperative markers associated with metabolic dysfunction and decreased kidney function were the main factors contributing to mortality risk.
These findings suggest that there is unclear clinical value in adding continuous, high-dimensional intraoperative hemodynamic and temperature data to ML prediction models that use routine postoperative data to identify mortality risk in patients undergoing cardiac surgery, the researchers concluded.
This study is the latest example of how researchers are investigating the usefulness of additional data for mortality prediction.
In July, researchers found that ML models incorporating social determinants of health (SDOH) data improve the prediction of in-hospital mortality among patients with heart failure, particularly Black patients. However, further research is needed to ensure that ML models incorporating SDOH data improve health outcomes without perpetuating biases or additional disparities in a clinical setting.