Machine learning characterizes cardiac function, drug response
New tool accurately quantifies contractile function and calcium handling, enabling the machine learning classification of cardiac disease and cardioactive drugs.
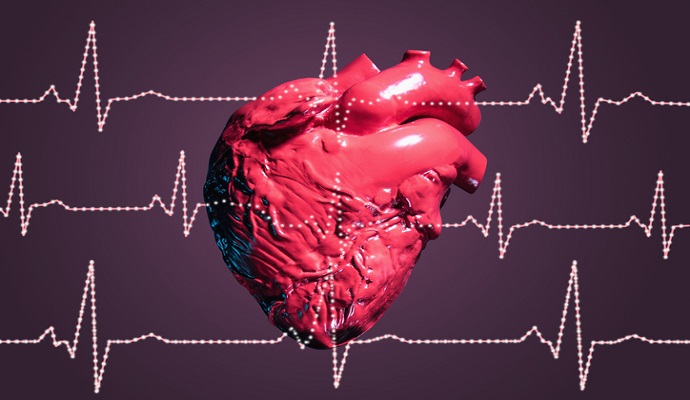
Source: Getty Images
- Columbia University researchers have developed a machine learning-based approach to assess cardiac function and drug response, according to a study published recently in IEEE Open Journal of Engineering in Medicine and Biology.
The research team indicated that understanding heart function and testing new drugs to treat cardiac diseases is both complex and time-intensive. The advent of cellular and engineered tissue models has helped reduce some of these burdens, but other challenges still remain.
Contractile response and calcium handling are key to assessing the heart’s function and physiology, and methods to test these in cellular and tissue models exist. However, the researchers underscored that such approaches are time-consuming, error-prone and require specialized equipment.
To address this, the research team sought to develop an approach to accurately quantify contractile function, calcium handling and force generation across in vitro cardiac models to facilitate analyses of function and drug response.
The researchers developed BeatProfiler – a suite of cardiac analysis tools that use machine learning – to characterize heart disease and dose-dependent drug response. The software is designed to automate heart cell analysis using video data, allowing the tools to measure contractility and other indicators of cardiac function.
By integrating multiple tools, the BeatProfiler system aims to make analyses more efficient while reducing the chance for errors.
“This is truly a transformative tool,” said project leader Gordana Vunjak-Novakovic, PhD, university professor and the Mikati Foundation Professor of Biomedical Engineering, Medical Sciences, and Dental Medicine at Columbia, in a news release. “It’s fast, comprehensive, automated, and compatible with a broad range of computer platforms so it is easily accessible to investigators and clinicians.”
The method’s development was spurred by the clinical need to diagnose heart diseases and tailor treatments more effectively. Doing so requires researchers to be able to test new potential drugs on in vitro cardiac models.
BeatProfiler is set to advance these efforts by allowing research teams to quantify the function of heart muscle cells known as cardiomyocytes, which is critical for studies investigating genetic cardiomyopathies, immune-mediated inflammation, cosmic radiation and drug discovery.
When tested, the system outperformed existing tools, analyzing cardiomyocyte function accurately and reliably without sacrificing efficiency. The suite of tools was able to detect subtle shifts in engineered heat tissue force response that other tools may miss up to 50 times faster than those tools, in some cases.
BeatProfiler’s performance helped the researchers to distinguish between different diseases and severity levels while also efficiently testing drugs that impact heart function.
“This level of analysis speed and versatility is unprecedented in cardiac research,” said lead author Youngbin Kim, a PhD candidate in Vunjak-Novakovic’s lab at Columbia Engineering. “Using machine learning, the functional measurements analyzed by BeatProfiler helped us to distinguish between diseased and healthy heart cells with high accuracy and even to classify different cardiac drugs based on how they affect the heart.”
The software is open-source and freely available to researchers.
Moving forward, the Columbia team will work to further validate the system and expand it for use in other cardiac research applications. Eventually, the researchers hope to adapt BeatProfiler for use in pharmaceutical settings to accelerate candidate drug testing.
Artificial intelligence (AI) is also advancing cardiovascular research in other areas.
Last week, a multi-institutional team of researchers described how an AI-based video biomarker could help clinicians more accurately understand which patients are likely to develop or have worsening aortic stenosis.
The approach, known as Digital [aortic stenosis] Severity index (DASSi), uses echocardiographic signatures to stratify the risk of aortic stenosis development and progression.
The tool achieved high performance in both patients without the condition and those with mild or moderate forms of the condition at baseline.