Machine learning approach may help tailor precision medicine treatments
New machine learning tool uses data on individualized molecular interactions to predict how a patient’s immune system will respond to foreign cells.
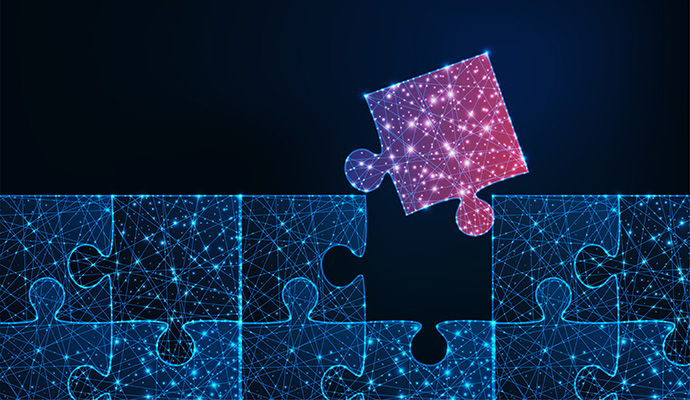
Source: Getty Images
- A research team from Arizona State University has developed a machine learning (ML) model capable of predicting whether a patient’s immune system will recognize pathogens and other foreign cells, according to a study published last week in Cell Systems.
The tool, called human leukocyte antigen (HLA)-Inception, is a convolutional neural network (CNN) that uses individualized molecular interaction information to understand how a group of proteins known as Major Histocompatibility Complex-1 (MHC-1) impact immune response.
MHC-1 proteins play a key role in the immune system’s ability to recognize foreign cells.
The proteins are found on the cell surface, where they bind and display foreign peptides for immune system recognition and attack. Being able to forecast which MHC-1s will bind with which peptides has significant potential to help advance immunotherapy and precision medicine.
However, the researchers indicated that MHC-1s are encoded by several genes with a large number of alleles, leading to thousands of different versions of these molecules being present in the human population. Each MHC-1 interacts with peptide fragments differently, making the development of a prediction model difficult.
To address this, the research team analyzed 5,821 MHC-1 alleles to reveal patterns in how these molecules prefer to bind with foreign peptides. Using electrostatic signatures present on the surface of the proteins, the researchers successfully identified 11 distinct MHC-1 classes.
The analysis further revealed that individuals with a diverse range of MHC-1s had a higher likelihood of surviving some cancer treatments.
These insights could be used to predict how an individual’s immune system is likely to respond to foreign cells.
“We are able to make predictions on pathological outcomes of patients, such as the survival against certain cancer medicines, based on the molecular details that a human is born with,” said lead author Abhishek Singharoy, PhD, an assistant professor in ASU’s School of Molecular Sciences, in a news release. “Now with this tool, something that was taking days only takes seconds.”
“This is impactful research with ramifications beyond the limits of academia,” Singharoy continued. “Our technique now is the fastest out there.”
The research team further emphasized that their predictive model may have utility in personalizing treatment plans for conditions like cancer.
“The continued integration of machine learning in health care will help de-risk and personalize treatments,” said Eric Wilson, PhD, a postdoctoral fellow at Icahn School of Medicine at Mount Sinai who contributed to the research. “Machine learning and AI can improve the accessibility of new treatments to a broader cohort of patients by negating the need for costly experiments to determine candidacy.”
The application of AI- and ML-based tools in precision medicine studies continues to grow as researchers explore the potential of these rapidly advancing technologies.
In March, researchers from Washington University School of Medicine in St. Louis detailed the development of a deep learning (DL) approach to predict which non-small cell lung cancer (NSCLC) patients are likely to experience brain metastasis.
Brain metastases occur in a significant portion of these patients, but the research team underscored that there are currently no reliable means to identify those at high risk.
To combat this, the researchers trained a DL algorithm to forecast brain metastasis risk using histopathology images of patients’ lungs. The tool successfully identified abnormal imaging features that a pathologist might miss and outperformed clinicians when tasked with flagging brain metastasis risk.