Harvard, Stanford Develop Self-Supervised AI to Detect Disease Via X-ray
Researchers from Harvard Medical School and Stanford University have developed an artificial intelligence model that doesn’t rely on human annotations of X-rays to learn to detect disease.
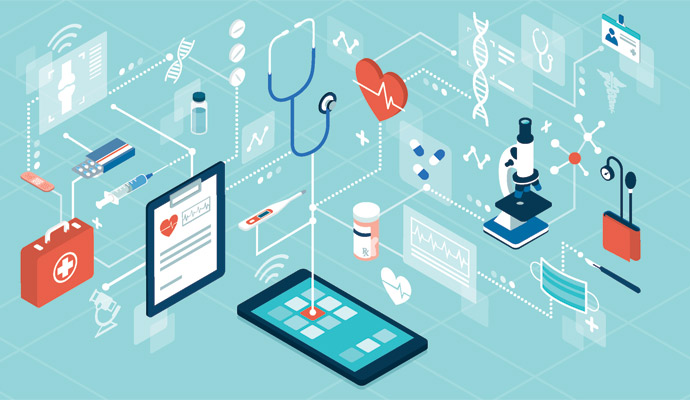
Source: Getty Images
- Harvard Medical School (HMS) and Stanford University researchers have developed an artificial intelligence (AI) tool that can detect disease within chest radiographs using natural language processing (NLP)-based clinical reports rather than relying on human annotations to “learn.”
Using AI to improve medical imaging is not new, but many challenges to AI use in this area keep it limited to a handful of clinical applications. One of these challenges is the burden of human annotation.
To “learn” to detect disease or other anomalies in medical images, AI models must be trained using relevant imaging data. However, to know what in the image is clinically important for the task it has been assigned, the AI must be trained using images that human clinicians have annotated.
The massive amount of data and annotations necessary for model training requires significant human effort. Researchers must take time to find clinical experts willing to annotate the images, instruct them in how the images must be annotated for that study’s purposes, and potentially compensate each annotator in some way. These are in addition to actually annotating the images, which can be a laborious process for annotators.
These hurdles can limit or slow researchers’ progress when developing or evaluating an AI imaging model. However, the model developed by HMS and Stanford, known as CheXzero, has shown that it can accurately detect disease within chest radiographs by relying on clinical reports generated by NLP rather than annotations made by humans.
The model is self-supervised, meaning that it trains itself to learn one part of the input from another part. Self-supervised learning (SSL) algorithms are a type of machine learning (ML) technique designed to address the issue of over-dependence on labeled data. In many real-world scenarios, researchers struggle to collect and label the amount of high-quality data they need. SSLs provide a low-cost, scalable alternative.
“We’re living the early days of the next-generation medical AI models that are able to perform flexible tasks by directly learning from text,” said study lead investigator Pranav Rajpurkar, PhD, assistant professor of biomedical informatics in the Blavatnik Institute at HMS, in the press release. “Up until now, most AI models have relied on manual annotation of huge amounts of data—to the tune of 100,000 images—to achieve a high performance. Our method needs no such disease-specific annotations.”
When they tested their model in a study published by Nature Biomedical Engineering this week, researchers found that the model was not only highly accurate when compared to three other models, but it also performed similarly to three radiologists.
“CheXzero shows that accuracy of complex medical image interpretation no longer needs to remain at the mercy of large labeled datasets,” said study co-first author Ekin Tiu, an undergraduate student at Stanford and a visiting researcher at HMS, in the press release. “We use chest X-rays as a driving example, but in reality CheXzero’s capability is generalizable to a vast array of medical settings where unstructured data is the norm, and precisely embodies the promise of bypassing the large-scale labeling bottleneck that has plagued the field of medical machine learning.”