Deep Learning Accurately Predicts Antihypertensive Treatment Success
A deep learning model can predict the likelihood of antihypertensive treatment success for individuals, which could help personalize hypertension management.
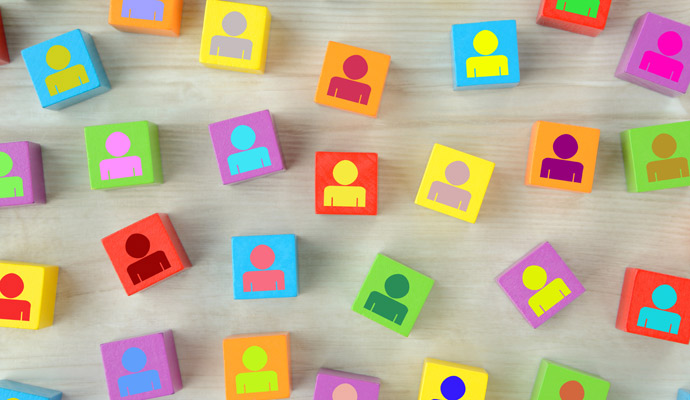
Source: Getty Images
- Researchers have developed a deep neural network-based model to predict the most successful antihypertensive treatment for an individual, according to a study published recently in Mayo Clinic Proceedings: Digital Health.
Hypertension is a major risk factor for a host of potentially life-threatening cardiovascular and other diseases, such as coronary disease, valvular heart disease, cardiac arrhythmia, renal failure, and cerebral stroke.
Effectively managing hypertension is key to reducing adverse outcomes, but the researchers noted that clinical guidelines for antihypertensive drug therapy are developed using data from aggregated studies. The findings from these studies are typically generalized to the level of the drug class — demonstrating similarities in blood pressure reduction and long-term cardiovascular outcomes across drug regimens —but individual treatment response varies widely.
To optimize and individualize hypertension treatment in primary care, the researchers set out to design a deep learning (DL) model that could predict antihypertensive treatment success at the patient level.
The tool was trained using data from 16,917 patients newly diagnosed with hypertension in Mayo Clinic’s primary care practices between January 1, 2005 and December 31, 2021.
Eligibility criteria for study participation included a diagnosis of primary hypertension, the presence of blood pressure and creatinine measurements taken before antihypertensive treatment, treatment within 9 months of initial diagnosis, and at least one year of follow-up.
The primary outcome evaluated in the study was the model’s performance on the task of predicting the likelihood of antihypertensive treatment success one year from the start of treatment. Antihypertensive treatment success was defined in the study as “achieving blood pressure control with no moderate or severe adverse effects.”
The model was then validated on a cohort of 1,000 patients.
Of the 16,917 patients in the training dataset, approximately 33.8 percent achieved blood pressure control without moderate or severe adverse effects for at least a year after starting treatment. The most common treatment used in this cohort was angiotensin-converting enzyme inhibitor, with an average success rate of 39.1 percent. The most successful treatment was angiotensin-converting enzyme inhibitor-thiazide combination, with 44.4 percent average success.
The deep neural network-based model achieved high performance, with a precision of 51.7 percent, recall of 44.4 percent, and F1 score of 47.8 percent when predicting individualized antihypertensive treatment success.
When compared with the Eighth Joint National Committee hypertension guidelines, actual clinician practice on the validation aligned 77.9 percent, while the model agreed with the guidelines 95.7 percent of the time.
These results led the research team to conclude that a deep learning tool has the potential to accurately predict the likelihood of antihypertensive treatment success, which could enhance personalized hypertension management.
Artificial intelligence (AI) and machine learning (ML) tools have also been applied to other cardiovascular conditions.
In November, research presented at the American Heart Association (AHA) Scientific Sessions showed that AI and deep learning may help flag heart disease and cardiovascular event risk.
In the first study, researchers compared the ability of primary care professionals using standard stethoscopes with that of an AI program using digital stethoscope data to detect potential heart valve disease. The AI significantly outperformed clinicians in this task, flagging 22 people with previously undiagnosed moderate-or-greater heart valve disease.
The second study investigated whether a deep learning tool could accurately determine patient risk of cardiovascular disease events by evaluating eye images of people with prediabetes and Type 2 diabetes. Following the 11-year study period, 12.5 percent of participants experienced a cardiovascular event, and the model was able to accurately classify participants into low-, moderate-, and high-risk groups.