ML Provides Personalized Hypertension Treatment Recommendations
A machine learning model may help clinicians maximize the effectiveness of hypertensive medications by making patient-specific treatment recommendations.
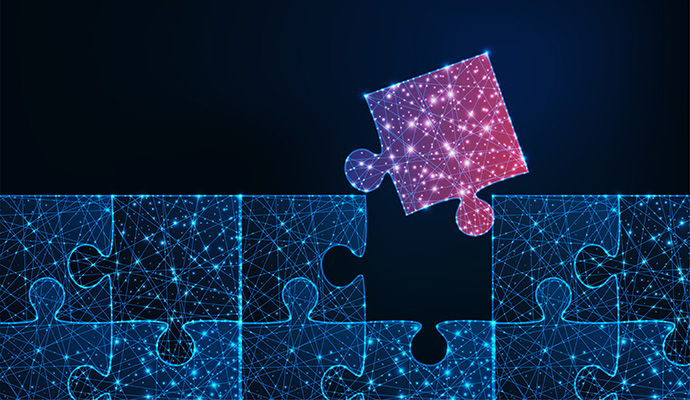
Source: Getty Images
- Boston University (BU) researchers have co-developed a machine learning (ML) model designed to provide personalized hypertension treatment recommendations and assist clinicians with choosing which hypertensive medication may be the best match for their patients.
In the study describing the model, published recently in BMC Medical Informatics and Decision Making, the researchers explained that prescribing the most effective medication for patients with hypertension can be a challenge. Genetic factors, comorbidities, and other patient traits can lead to various treatment responses depending on the antihypertensive drug prescribed.
Personalized hypertension treatment has the potential to address these hurdles, but such an approach has not been widely adopted in clinical care.
This led the researchers to create an ML-based clinical decision support tool aimed at giving care teams real-time hypertension treatment suggestions to help reduce systolic blood pressure more effectively than through current standard of care methods.
The model uses patient-specific characteristics, such as medical history, demographics, vital signs, and test results from EHRs to provide clinicians with a ‘custom’ hypertension prescription.
“This is a new machine learning algorithm leveraging information in electronic health records and showcasing the power of AI in healthcare,” said Ioannis Paschalidis, PhD, a BU College of Engineering distinguished professor and member of the research team, in the news release detailing the study. “Our data-driven model is not just predicting an outcome, it is suggesting the most appropriate medication to use for each patient.”
The model uses an individual patient’s profile to generate a list of suggested medications, which also includes the associated probability of success for each drug based on that patient’s information and how effective the medication is in a group of similar patients.
“Our goal is to facilitate a personalization approach for hypertension treatment based on machine learning algorithms [and] seeking to maximize the effectiveness of hypertensive medications at the individual level,” Paschalidis stated.
To develop the model, the researchers pulled de-identified data from the medical records of 42,752 patients at Boston Medical Center (BMC) from 2012 to 2020.
Patients were sorted into groups based on clinically relevant characteristics, including demographics, medical history, and past blood pressure readings. From there, the data were fed to the ML model and three additional algorithms designed to predict appropriate hypertension treatment plans.
The effectiveness of each model was then compared to that of the current standard of care.
The ML model performed 7.08 percent better than the second-best algorithm and achieved a 70.3 percent larger reduction in systolic blood pressure than the standard of care.
The ML model also highlighted the value of reducing or stopping antihypertensive drugs for some patients who were already taking multiple medications.
“These advanced predictive analytics have the ability to augment a clinician’s decision making and to have a positive impact on the quality of care we deliver, and therefore the outcomes for our patients,” explained Rebecca Mishuris, MD, Mass General Brigham’s chief medical information officer who previously served as an assistant professor at BU Chobanian & Avedisian School of Medicine. “This is an important first step that shows that these models actually perform better than standard of care, and could help us be better doctors.”
The study also underscored how artificial intelligence (AI) models can help improve care quality and personalized medicine approaches.
“Using data from the diverse patient population of Boston Medical Center, this model provides the opportunity to tailor care for underrepresented populations, with individualized recommendations to improve outcomes for these patients,” said Nicholas J. Cordella, MD, an assistant professor at BU Chobanian & Avedisian School of Medicine. “Personalized medicine and models like this are an opportunity to better serve populations that aren’t necessarily well represented in the national studies or weren’t taken into account when the guidelines were being made.”