Decentralized AI Connects Globally Distributed, Poor-Quality Medical Data
Researchers have developed a decentralized algorithm that can be used to train AI on data that is distributed globally, without having to move the data to a central location.
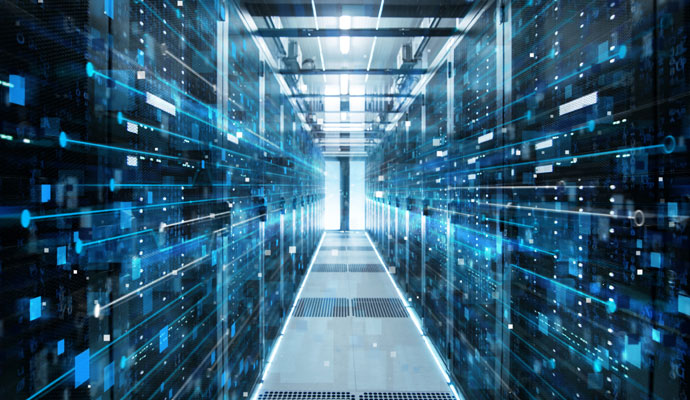
Source: Getty Images
- A new study published in Scientific Reports shows that a federated learning algorithm designed to train artificial intelligence (AI) on globally distributed, decentralized, and poor-quality medical data – without the use of data sharing — achieves better results than traditional, centralized AI training with real-world, poor-quality data.
Training AI on large datasets is necessary to ensure their accuracy and lower the potential for bias, but data sharing is a major obstacle in industries such as healthcare because of privacy laws. Sharing data can also be a challenge because of the way the data is stored and data quality, which is often low because of normalization or completeness issues.
Federated learning, a type of machine learning (ML), can help address some of these challenges. Federated learning approaches allow AI algorithms to be trained across multiple servers or devices, which are usually distributed across various locations, without the need to exchange, share, or move that data in any way. Because the data is not shared to a central server to train the AI, as it would be using traditional training methods, healthcare organizations can utilize AI technologies without endangering patient privacy.
In this study, the federated learning model, Decentralized AI Training Algorithm (DAITA), was used to evaluate the performance of a decentralized approach to training healthcare AI compared to a traditional, centralized approach. DAITA works by moving the AI it is training to the location of the data, rather than moving the data to a centrally located AI. This allows for globally distributed data to be used for AI training, which can enhance both the size and diversity of the data.
Using this method, only the general, abstract learnings of the AI are shared, rather than individual datasets. To further protect patient data, the AI models being moved to each data location for training are designed so that they cannot be reverse engineered to reveal that data.
For the study, researchers tested the models using a non-medical dataset containing intentionally distorted, synthetic data, also known as noise, and a medical dataset. This highlights the generalizability of a given model across multiple locations, which is key to a federated learning model’s viability. Data quality within these sets also varied, as it would in many healthcare datasets, to further evaluate the models’ performances.
Overall, DAITA’s performance was found to be comparable to that of a centralized approach when using the non-noisy medical dataset. When considering noisy or poor-quality data, however, DAITA is found to outperform the centralized training approach in terms of accurately training AI algorithms.
These results showcase the potential of federated learning to help health systems utilize AI while protecting patient privacy. However, further research is needed to validate federated learning models for real-world use.