ML Model Detects Patients at Risk of Postoperative Issues
Pennsylvania-based researchers used medical records to develop and validate an ML model to predict patients at risk of complications after surgery.
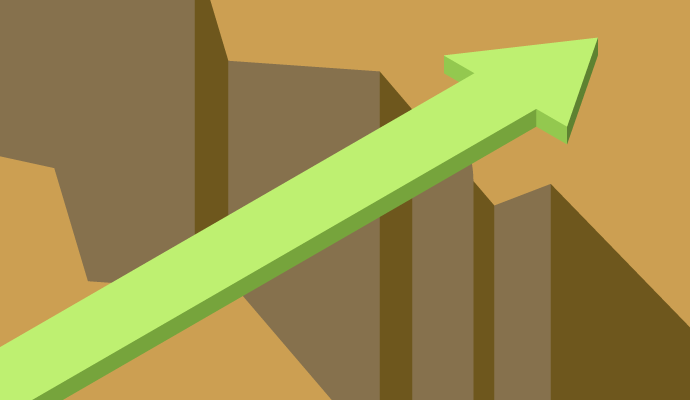
Source: Getty Images
- To enhance the process of identifying medical issues in patients after operations, two researchers from the University of Pittsburgh and UPMC created a machine-learning (ML) model trained on data from more than 1.25 million surgical patients.
According to the press release, complications after surgery are a major cause of death. Before the COVID-19 pandemic, it was the third leading cause of death, killing about 4.2 million people yearly.
To save lives while lowering costs, two researchers created an ML model to support the process of identifying complications post-operation. The two researchers are Aman Mahajan, MD, PhD, chair of anesthesiology and perioperative medicine, Pitt School of Medicine, and director of UPMC Perioperative and Surgical Services, and Oscar Marroquin, MD, chief health care data and analytics officer at UPMC.
“Improving overall health of patients prior to surgery through prehabilitation can go a long way in improving outcomes for high-risk patients,” said Mahajan in a press release. “However, identifying high-risk patients can be challenging for busy clinicians, who have to integrate the wealth of health data available and frequently perform additional testing and clinical assessments. We wanted to build an easy-to-use model that provides the health care team with an automated and accurate risk assessment quickly using existing data in the electronic health record.”
The process of building the model consisted of training the algorithm to learn from the records of over 1.25 million patients. The algorithm was focused on mortality and the presence of a cardiac event post-surgery. Following this, the researchers validated the model on data from another 200,000 patients who had surgery at UPMC.
Following the validation process, researchers deployed the model to 20 UPMC hospitals. At the start of each day, it reviews the records of patients coming in for surgery. As it does this, it highlights those that are high risk. If need be, patients can be directed to 'prehabilitation' services, such as a referral to the UPMC Center for Perioperative Care, or be advised to make healthier decisions prior to surgery.
Following its creation and implementation, researchers compared the model's efficacy to other methods. After comparing their ML model to the American College of Surgeon’s National Surgical Quality Improvement Program (ACS NSQIP), they found that the tool performed better and more efficiently.
“We designed our model with the health care worker in mind,” said Marroquin in the press release. “Since our model is completely automated and can make educated predictions even if some data are missing, it adds almost no additional burden to clinicians while providing them a reliable and useful tool.”
Further, researchers aim to use the model to predict conditions such as sepsis and respiratory issues.
ML models are commonly applied to surgical practices in an effort to make processes more efficient.
Research from December 2022 indicated that the use of preoperative data and intraoperative hemodynamic monitoring data in an ML model was assistive in predicting the need for massive transfusion.
To address complications associated with uncontrolled intraoperative hemorrhage, massive transfusion is critical. But preparing blood products for massive transfusions is time-consuming and requires multiple medical personnel. Due to this, early prediction of the need for massive transfusion is necessary.
Researchers considered preoperative and intraoperative hemodynamic monitoring data to build a real-time prediction model for massive transfusion. This led to high accuracy in predicting potential postoperative massive transfusions during surgery, leading to early intervention when needed.