Cedars-Sinai Develops AI to Improve Accuracy of Heart Condition Detection
Researchers from Cedars-Sinai have developed artificial intelligence models that improve accuracy in predicting heart attacks and detecting coronary artery disease.
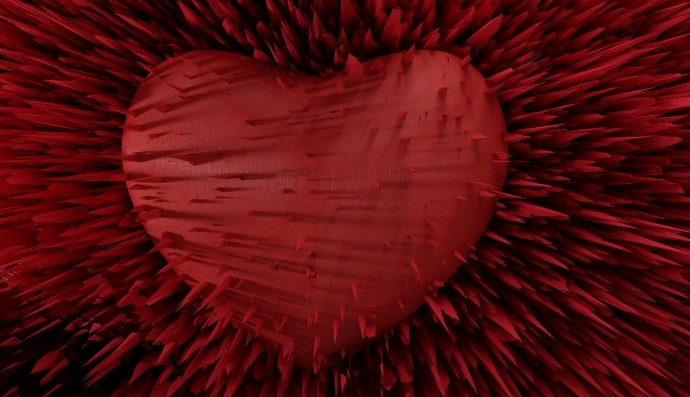
Source: Getty Images
- Researchers in the Division of Artificial Intelligence in Medicine at Cedars-Sinai have developed artificial intelligence (AI) models that improve quality and accuracy in heart imaging, predicting heart attacks and other major adverse cardiac events, and diagnosing coronary artery disease.
The first tool, described in a study published in The Journal of Nuclear Medicine in September, leverages AI to improve the diagnostic accuracy of single-photon emission computerized tomography (SPECT) imaging, which the press release notes is commonly used to diagnose coronary artery disease.
According to the study, SPECT imaging requires attenuation correction, which helps reduce artifacts in heart images. These artifacts can make the images more difficult to read or less accurate, but attenuation correction requires an additional CT scan and hybrid SPECT/CT equipment.
The additional scan time, radiation, and limited availability of these devices mean that attenuation correction is only performed on a small number of scans, despite the evidence indicating that the method improves coronary artery disease diagnosis.
To address this, a team of Cedars-Sinai researchers set out to develop an AI tool that could eliminate the need for hybrid scanners. Their model, known as DeepAC, uses deep learning to generate corrected SPECT images without hybrid scanners, similar to techniques used to create “deep fake” images and videos.
To gauge the tool’s diagnostic accuracy, they compared non-corrected SPECT images, advanced hybrid SPECT/CT images, and new DeepAC-corrected images. The team found that the AI-generated images were near the same quality and allowed similar diagnostic accuracy as those obtained from traditional scanners.
“This AI model was able to generate DeepAC images in a fraction of a second on standard computer software and could readily be implemented in clinical workflows as an automatic pre-processing step,” said Piotr Slomka, PhD, director of Innovation in Imaging at Cedars-Sinai and a research scientist in the Division of Artificial intelligence in Medicine and the Smidt Heart Institute, in the press release.
A second study, published this month in the Journal of American College of Cardiology: Cardiovascular Imaging, showcased how another Cedars-Sinai AI tool could predict heart attacks and other major adverse cardiac events.
Like DeepAC, this tool leverages heart images to generate insights. The model was trained using 20,000 SPECT scans depicting heart perfusion and motion for each patient in the database. The tool was designed to incorporate visual explanations for the clinicians from the images, highlighting images with areas that contribute to a high risk of adverse events.
The researchers tested the model in two separate sites with more than 9,000 scans. They found that it predicted patient risk more accurately than the software programs currently used in the clinic.
“In the first study, we were able to demonstrate that AI can be used to perform important image corrections without the need for expensive scanners,” Slomka said. “In the second, we show that the existing images can be utilized in a better way — predicting patient risk of heart attack or death from images, and highlighting the heart features which indicate that risk, to better inform clinicians about coronary artery disease.”
The press release states that moving forward, the researchers aim to evaluate these AI approaches further to explore how they may be integrated into clinical software and routine patient care.
These tools are part of a growing toolkit of AI solutions that clinicians are utilizing in clinical settings.
In May, Geisinger shared that its clinicians had adopted an AI tool known as HeartFlow Analysis, which allows care teams to use data from patients’ coronary CT scans to generate 3D models of their coronary arteries. These models allow clinicians to assess patients for coronary artery disease without performing unnecessary invasive procedures in the operating room or tests in the emergency department.