AI Smartphone Tool Accurately Diagnoses Pediatric Ear Infections
An artificial intelligence-driven smartphone app may help diagnose childhood ear infections and guide appropriate antibiotic treatment.
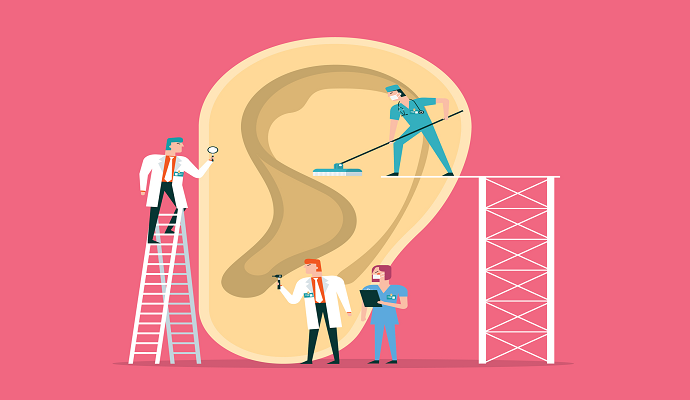
Source: Getty Images
- Researchers from the University of Pittsburgh (Pitt) and UPMC have developed a smartphone application that leverages artificial intelligence (AI) to diagnose ear infections, specifically acute otitis media (AOM), in pediatric patients, according to a study published today in JAMA Pediatrics.
The research team emphasized that diagnosing AOM in primary care settings can be a challenge, as discerning between it and other ear conditions requires intensive training. This hurdle is compounded by the fact that AOM is common in children and requires treatment with antibiotics; clinicians’ struggle to differentiate between AOM and other conditions could lead to unnecessary antibiotic use.
“Acute otitis media is often incorrectly diagnosed,” explained senior author Alejandro Hoberman, MD, professor of pediatrics and director of the Division of General Academic Pediatrics at Pitt’s School of Medicine and president of UPMC Children’s Community Pediatrics, in a press release shared with HealthITAnalytics. “Underdiagnosis results in inadequate care and overdiagnosis results in unnecessary antibiotic treatment, which can compromise the effectiveness of currently available antibiotics. Our tool helps get the correct diagnosis and guide the right treatment.”
The smartphone app works by analyzing short videos of the eardrum taken using an otoscope, which could help capture subtle visual findings key to accurately diagnosing AOM. The researchers noted that AOM is often confused with otitis media with effusion, a condition that typically doesn’t involve bacteria or benefit from the use of antibiotics.
“The eardrum, or tympanic membrane, is a thin, flat piece of tissue that stretches across the ear canal,” said Hoberman. “In AOM, the eardrum bulges like a bagel, leaving a central area of depression that resembles a bagel hole. In contrast, in children with otitis media with effusion, no bulging of the tympanic membrane is present.”
The tool is designed to capture eardrum features like shape, color, translucency, and position to assist clinicians with diagnosis.
To develop the app, the research team gathered and annotated 1,151 training videos looking at the tympanic membranes of 635 children who received outpatient care at UPMC from 2018 to 2023. Each video was reviewed by two clinicians with expertise in AOM, who categorized the videos as either AOM or no AOM.
Two AI models – a deep residual-recurrent neural network and a decision tree network – were trained on 921 videos from this set, and their performance was assessed using the remaining 230 videos.
Both models demonstrated high performance, achieving similar accuracy. The finalized deep residual-recurrent neural network algorithm reached a sensitivity of 93.8 percent and a specificity of 93.5 percent, whereas the decision tree model demonstrated 93.7 percent sensitivity and 93.3 percent specificity.
The research team underscored that previous studies have found clinicians’ accuracy in diagnosing AOM ranges from 30 to 84 percent.
“These findings suggest that our tool is more accurate than many clinicians,” Hoberman stated. “It could be a gamechanger in primary health care settings to support clinicians in stringently diagnosing AOM and guiding treatment decisions.”
Aside from its diagnostic potential, the researchers also highlighted the tool’s potential for use in patient and provider education.
“Another benefit of our tool is that the videos we capture can be stored in a patient’s medical record and shared with other providers,” said Hoberman. “We can also show parents and trainees — medical students and residents — what we see and explain why we are or are not making a diagnosis of ear infection. It is important as a teaching tool and for reassuring parents that their child is receiving appropriate treatment.”
As excitement around the use of healthcare AI tools grows, significant research has been dedicated to how these technologies can improve diagnostics and clinical decision support.
However, some researchers have raised concerns about how these tools and the data collection needed to drive their adoption will impact vulnerable populations like children.
In a November interview with HealthITAnalytics, one clinical AI researcher from Stanford University detailed the development of the ACCEPT-AI framework – a set of guidelines for the safe inclusion of pediatric data in AI and machine learning research – and discussed how researchers and regulators can protect children’s interests in the context of such research.