Artificial Intelligence Models May Enhance Immunotherapy Treatments
Research findings from Cleveland Clinic and IBM demonstrated how AI models can better show how immune systems recognize threats, which may improve immunotherapies.
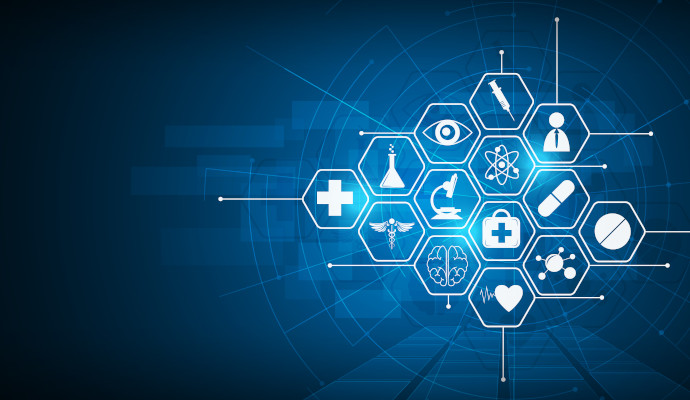
Source: Getty Images
- Researchers from Cleveland Clinic and IBM have found that artificial intelligence (AI) models can help provide insights into how antigen peptides interact with immune cells, which could be used to identify more effective immunotherapy targets, according to a study published recently in Briefings in Bioinformatics.
Designing immunotherapies relies on identifying antigens – foreign substances commonly found in tumors, bacteria, and viruses that trigger an immune response – and using them to attack infected or cancerous cells.
However, the process of how antigen peptides interact with immune cells is based on features of the cell’s surface. The large number of variables that impact how immune systems recognize these features and use that information to attack infected cells is not yet well understood, and research progress has been hampered as a result.
Models to identify these variables exist, but they rely on standard computing, making the process time-consuming and difficult. These challenges render the models severely limited at best and inaccurate at worst.
To overcome these hurdles, the researchers turned to AI models.
“In the past, all our data on cancer antigen targets came from trial and error,” said Timothy Chan, MD, PhD, chair of Cleveland Clinic’s Center for Immunotherapy and Precision Immuno-Oncology and Sheikha Fatima Bint Mubarak Endowed Chair in Immunotherapy and Precision Immuno-Oncology, in a press release. “Partnering with IBM allows us to push the boundaries of artificial intelligence and health sciences research to change the way we develop and evaluate targets for cancer therapy.”
The research team trained unsupervised and supervised AI models to predict antigen peptide immunogenicity – the ability of cells and tissues to provoke an immune response.
In doing so, the researchers found that unsupervised AI could identify subtle features that drive immunogenicity, and that both unsupervised and supervised models highlighted determinants of immunogenicity.
By accounting for fluctuations in molecular shape over time, the AI can show how immune cells recognize target antigens. Using these tools, researchers could gain a better understanding of which processes to target with immunotherapy treatments.
In turn, this knowledge could help researchers to design improved immunotherapies in the future.
The study is the first peer-reviewed publication from Cleveland Clinic and IBM’s Discovery Accelerator partnership, launched in 2021 to bolster healthcare and life sciences research through the use of AI, high-performance computing, and quantum computing.
“These discoveries are an example of what makes this partnership successful – combining IBM’s cutting-edge computational resources with Cleveland Clinic’s medical expertise,” stated Jeff Weber, PhD, senior research scientist at IBM. “These findings resulted from a key collaboration between everyone from a world-class expert in cancer immunotherapy to our physics-based simulation and AI experts. Collaboration when combined with innovation has terrific potential.”
Cleveland Clinic is also leveraging other advanced technologies to improve patient care.
In December, Jarrod Dalton, PhD, director of Cleveland Clinic’s Center for Populations Health Research, sat down with HealthITAnalytics for a conversation about the health system’s collaboration with MetroHealth to develop a digital twin-based, neighborhood-focused model to reduce disparities in their patient populations.
The Digital Twin Neighborhoods project will synthesize de-identified health information pulled from electronic health records (EHRs) to generate digital replicas of real communities served by the two health systems.
By incorporating social, biological, geographical, and social determinants of health (SDOH)-related information, the project leads hope to gain insights into place-based health and the associated disparities, which will be used to drive health equity initiatives.