Understanding the Basics of Clinical Decision Support Systems
How can clinical decision support systems deliver patient safety and care quality improvements while ensuring health IT usability?

Source: Thinkstock
- Clinical decision support systems are quickly becoming essential tools for healthcare providers as the volume of available data increases alongside their responsibility to deliver value-based care.
Reducing clinical variation and duplicative testing, ensuring patient safety, and avoiding complications that may result in expensive hospital readmissions are top priorities for providers in the modern regulatory and reimbursement environment – and harnessing the hidden insights of big data is essential for achieving these goals.
Clinical decision support (CDS) tools are designed to help sift through enormous amounts of digital data to suggest next steps for treatments, alert providers to available information they may not have seen, or catch potential problems, such as dangerous medication interactions.
“The amount of information we need to understand is getting so untenable that it’s unreasonable to expect the average clinician to integrate all of it into their decision-making effectively and reliably,” said Dr. Joe Kimura, Chief Medical Officer at Atrius Health.
“If we really want to make sure every human being gets great care, then you have to make sure that you’re assisted by technology.”
CDS tools are often integrated into the electronic health record (EHR) to streamline workflows and take advantage of existing data sets, although many organizations are still facing significant challenges when it comes to creating intuitive, user-friendly, and effective protocols for alarms, alerts, and decision-making pathways.
Alert fatigue and clinical burnout are common byproducts of poorly implemented clinical decision support features that overwhelm users with unimportant information or frustrating workflow freezes that require extra clicks to circumvent.
Alert and alarm fatigue consistently make industry lists of health IT hazards and patient safety, with experts like ECRI Institute warning that users can quickly become desensitized to critical alerts when inundated with too many low-priority popups, sounds, or messages.
In 2016, a study published in JAMA Internal Medicine found that EHR users spend more than an hour each day processing notifications. Primary care providers receive an average of 76.9 notifications every day.
While not all of those notifications are generated by clinical decision support systems – many came from lab results, pharmacies, or other physicians – adding CDS alerts to an inbox already overflowing with input can do more harm than good.
How can healthcare organizations create valuable and effective clinical decision support tools that improve care quality while avoiding the common pitfalls of poorly deployed notifications and inefficient workflow strategies?
What is clinical decision support?
Clinical decision support is any tool that provides clinicians, administrative staff, patients, caregivers, or other members of the care team with information that is filtered or targeted to a specific person or situation.
CDS is intended to improve care quality, avoid errors or adverse events, and allow care team members to be more efficient.
HealthIT.gov notes that CDS can include a wide variety of decision-making aids, such as “computerized alerts and reminders to care providers and patients; clinical guidelines; condition-specific order sets; focused patient data reports and summaries; documentation templates; diagnostic support, and contextually relevant reference information, among other tools.”
Clinical guidelines and diagnostic support frameworks do not have to be digital, but the modern definition of CDS tends to focus on health IT applications, modules, or analytics that leverage an organization’s big data assets.
For providers participating in Stage 2 of the EHR Incentive Programs, the definition of CDS is “HIT functionality that builds upon the foundation of an EHR to provide persons involved in care processes with general and person-specific information, intelligently filtered and organized, at appropriate times, to enhance health and health care.”
But the Office of the National Coordinator declined to make the definition any more precise, in part to encourage the development of new CDS tools that could help support population health management, precision medicine, value-based care, patient safety, and operational efficiencies.
“CDS is not only for doctors or nurses, but also for support staff, patients, and other caregivers,” explains the CMS eHealth University.
“For instance, some practices have used ‘return to clinic’ reminders available in their EHRs to remind front desk staff to proactively call patients due for routine screenings to remind them of upcoming appointments and/or explain pre-visit preparations such as fasting, outside lab work, etc. CDS delivered to patients could take the form of detailed medication instructions, home management tips, or dietary guidelines.”
Ultimately, CMS says, clinical decision support can include any health IT system, workflow, or process intended to deliver:
- the right information (evidence-based guidance, response to clinical need)
- to the right people (entire care team – including the patient)
- through the right channels (e.g., EHR, mobile device, patient portal)
- in the right intervention formats (e.g., order sets, flow-sheets, dashboards, patient lists)
- at the right points in workflow (for decision making or action)
Hospitals have been particularly quick to try to apply these imperatives to their care processes.
By 2013, just under 73 percent of hospitals participating in meaningful use had adopted enough CDS capabilities to meet the core objective measure of “implement[ing] at least 5 clinical decision support interventions related to 4 or more clinical quality measures,” the ONC says.
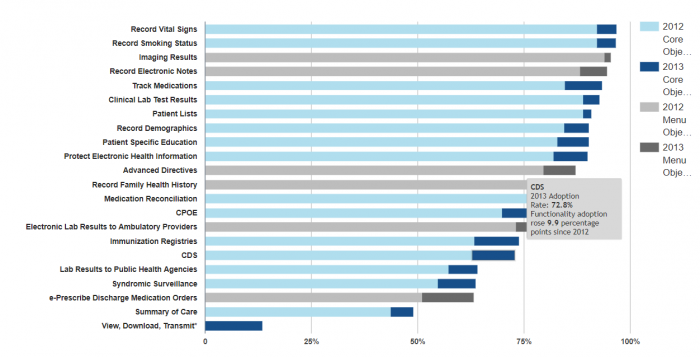
Source: ONC
Fifty-seven percent of eligible providers (EPs) had met their version of that goal in 2013, including providing reminders for interventions or screenings based on clinical guidelines.
The Difference Between Clinical Decision Support, Big Data Analytics
Top Clinical Decision Support System Companies by Ambulatory, Inpatient Settings
What are the use cases for CDS technology?
The basic principles of CDS can be applied to questions of patient care in an infinite number of ways, from the early detection of infection to delivering insights into highly personalized cancer therapies.
Some promising use cases from the provider community include:
- A hospital in Alabama decreased its sepsis mortality rates by 53 percent after implementing a computerized surveillance algorithm. Real-time analytics alerted providers to new diagnoses of sepsis or worsening vital signs, and also provided reminders about best practices for treating patients with the deadly condition.
- Mayo Clinic is currently employing a CDS tool that helps nurses deliver complete and accurate phone screenings of patients seeking advice or appointments. The computerized decision software guides triage nurses through a series of standardized questions based on current care guidelines, ensuring that they do not miss important information about the patient’s health.
- Harding University and Unity Health-White County Medical Center found that combining a CDS system with genetic testing data could reduce hospital readmissions by 52 percent and reduce ED visits by 42 percent. Conducting drug-drug and drug-gene interaction testing on high-risk patients helped produce an estimated cost savings of more than $4300 per capita.
- A clinical decision support application for patients presenting with head injuries, developed by Yale and the Mayo Clinic, uses industry guidelines to provide information to patients while evaluating the severity of the injury. The application explains decision treatments to patients while potentially reducing the number of unnecessary CT scans, a high-cost test that is not always warranted.
- At a Department of Veterans Affairs site in Indiana, clinical decision support tools geared towards reducing unnecessary lab utilization helped decrease total test volume by 11.18 percent per year, generating cost savings of more than $150,000 without impacting care quality.
Additional areas where providers could benefit from CDS tools include:
- Completing drug dosing calculations
- Accessing drug formulary guidelines
- Using severity indexes for specific illnesses
- Tailoring order sets or templates for specific diseases
- Identifying reportable conditions based on EHR inputs
- Using time-triggered reminders for medication delivery or dosage changes
- Identifying needed preventive care screenings or care gaps (mammograms, colonoscopies, flu shots)
- Accessing filtered reference information or educational materials
CDS tools are increasingly leveraging machine learning and artificial intelligence to power sophisticated analytics. Machine learning algorithms can ingest large quantities of data, identify patterns, and return detailed results to users.
Machine learning tools have become particularly valuable for imaging analytics, Intent of Things analytics, and precision medicine.
At the University of Pennsylvania, machine learning powered a CDS tool that reduces the time to detect sepsis by 12 hours, which could be the difference between life and death for many individuals. The algorithm used data from more than 160,000 patients for training, and was validated on a sample of a further 10,000 individuals.
In one recent study from MIT, a deep learning tool uses bedside monitors, clinical notes, and other data sources to generate hourly predictions about ICU patients in order to give providers a head start on altering their care plans for highly complex patients.
The tool also delivers rationales for its recommendations – a key component of creating machine learning systems that providers can trust.
As machine learning and AI become more integral to the healthcare big data environment, more and more CDS tools are likely to be built upon these advanced analytics methodologies.
Turning Healthcare Big Data into Actionable Clinical Intelligence
Top 10 Challenges of Big Data Analytics in Healthcare
How can healthcare organizations implement CDS effectively?
No matter what the technical foundation, clinical decision support tools can easily turn from a blessing into a curse for clinicians.
Adding any new technology to a workflow can be a dicey proposition for organizations dealing with an epidemic of provider burnout, so leaders should take the time to carefully plan the rollout of any new CDS functionality.
The magnitude of frustration with the EHR workflow cannot be underestimated: one recent AMA study indicates that physicians are spending more time at the keyboard than they do with their patients.
Physician burnout is “a public health crisis,” declared leaders from many of the top provider advocacy and care delivery organizations in the country, citing inefficient practice environments, EHR technology, and excessive clerical work as major reasons why physicians are being driven out of the healthcare industry at an untenable rate.
In such a grim environment, organizations leaders should keep in mind that new CDS functions must reduce the need for frustrating interactions with the electronic health record, not increase the time spent in front of the screen without producing a measurable positive result.
There are ways to be successful, however – and the first steps involve acknowledging the clinical burnout elephant in the room.
Building a team and defining the project’s scope
CMS suggests that “starter conversations” around CDS implementation begin with an assessment of the organization’s cultural readiness to accept new decision support tools.
“CDS implementation and adoption cannot begin until it is clear that your organization is ready to use CDS for your selected clinical goals and priorities,” the agency says in a resource guide.
“Assessing this readiness is critical. To understand how well your organization can handle change (what type of change, how much, and over what time period) is a key aspect of readiness. The concept of “adaptive reserve” – what resources are available to plan for, adapt to, and reflect on major change in an organization – is helpful to consider.”
While many providers will have an immediately negative reaction to the idea of adding new steps to the workflow, Kimura points out that most clinicians are already benefiting from existing CDS tools.
“I don’t think there’s a doctor out there using an EHR that feels the medication-allergy cross-check is not helpful,” he said. “We used to turn to a clinical pharmacist and ask if there was anything we needed to worry about. The pharmacist would say, ‘Nope, you’re good,’ and we’d finish prescribing the medication.”
“Now the computer can do that, and it does it really well. That’s a good deal for us as clinicians, and an even better deal for our patients. I don’t know a single colleague who feels that getting [an] alert, just because it comes from a computer, would somehow diminish their ability to be a better physician.”
Reminding providers that some health IT advances have already produced positive impacts could help to soften their resistance to the idea of adding new items to their toolkit.
A collaborative approach to these conversations also helps, CMS adds. Physicians, nurses, administrators, and other technology users should be part of the discussion when identifying promising use cases and considering CDS vendors or implementation strategies.
Tapping clinical champions, including members of the nursing staff, could help to create a more receptive environment while developing a feedback pipeline to inform future efforts.
Effective clinical champions are tech-savvy members of the organization who are trusted by their peers, understand the technical and workflow challenges of their colleagues, can provide a balanced and informed perspective, and have strong interpersonal skills that can help them handle conflict, skepticism, or frustration.
During this part of the implementation process, organizations may wish to ask themselves:
- Do we have the resources to implement, update, and maintain clinical decision support tools?
- Is there resistance from clinical or administrative stakeholders? A lack of buy-in from executive leaders? How can their concerns be addressed?
- Has the case for change been explained effectively? Have we solicited feedback from stakeholders and truly taken their opinions on board?
- Does every member of the leadership team have a clear, well-defined role that they have the time and expertise to fulfill?
Creating a single source of truth
Many health IT projects fail because organizations are working off of different data, different visions for the outcome, or different ideas of how a tool will affect their daily operations.
When working with analytics, organizations must ensure that they have developed shared definitions for key elements, such as the suggested interval between cancer screenings for patients in different age brackets.
They should also agree upon what exactly constitutes a success: a ten percent increase in the number of shingles vaccines for patients over the age of 65, a two percent reduction in duplicated MRIs, or a savings of $10,000 on knee replacements for Medicare patients.
“Start simple and focus on an ‘easy win’ in both clinical goal and objectives, and corresponding CDS interventions,” CMS says.
“Make the early interventions easy to implement with outcomes that can be easily tracked. Add complexity to your interventions only after the practice gains more experience applying CDS strategies to improvement goals.”
Ensuring that all stakeholders are on the same page can reduce the possibility of conflict or finger-pointing when a benchmark is not achieved.
Organizations should also take the time to document workflows before a CDS intervention, as well as afterwards, to monitor productivity and ensure that leaders have more than anecdotal evidence to use when making future decisions.
“Keep in mind ‘upstream’ and ‘downstream’ activities that need to happen before and after physician-focused CDS for the intervention to have its intended effect,” CMS notes.
“For example, ensure that ample supplies of flu shots and nurse time are available to handle a desired uptick in flu vaccination rates in response to CDS focused on this objective.”
Working with health IT vendors to fine-tune CDS tools
Healthcare organizations should not only ensure that they understand the workflow impacts before rolling out a new function, but also that the CDS tool works as intended – and particularly that it works well for the use cases at hand.
Many CDS functionalities are created and deployed by EHR vendors as part of their product suites. While this is a benefit for organizations that do not have the in-house experience to develop their own proprietary tools, it also requires providers to have a strong working relationship with their vendor to ensure that the function meets the provider’s specific needs.
“The practice’s EHR supplier – and associated CDS knowledge-based content providers (drug-drug interaction checking, drug-allergy checking, reminders, order sets and templates) should make it as easy as possible for the practice to incorporate, validate updates, monitor, and ensure critical changes as needed to the CDS interventions,” CMS says.
“When communicating enhancement requests, it is best to identify the problems that the practice is facing – rather than propose a solution. Let the vendor assimilate and understand the problem deeply so that they can work with the practice to identify a good solution(s).”
Measure success to ensure continuous improvement
Collecting feedback after an implementation is just as important as getting stakeholder input before the go-live. Measuring the impacts on workflow, patient safety, care quality, and provider/patient satisfaction can help inform future efforts and make positive changes to the process.
Elaborating on information originally developed by HIMSS, CMS outlines several key performance indicators that can help organizational leaders understand the impact of a CDS tool:
- Health IT usability scores from end-users, compared to pre-implementation baseline
- Time-to-completion for specified tasks before and after CDS roll-out
- Rate at which end-users override alerts
- Adherence to clinical guidelines for selected care pathways
- Time-to-order for critical medications/procedures
- Increases or decreases in blood glucose control, lipid levels, preventive screening rates
- Reductions in duplicated or unnecessary laboratory tests
- Number of error reports, patient safety reports, or medication prescribing errors
Collecting qualitative feedback from providers is equally important, the guide states. “End-user feedback gathered through support structures during roll out should serve to identify initial challenges and lessons learned with the intervention,” the agency says.
“Formal and informal means of gathering end-user feedback – from short satisfaction questionnaires, email based assessment forms, to hallway and lunchroom conversations – may be used to assess end-user experiences. Consider using anonymous means of feedback as well; a suggestion box may serve to help collect input from otherwise silent end-users.”
Recognizing that a CDS deployment is not only a technical project is an important component of success. Clinical decision support tools can only improve patient safety and care quality if providers accept the addition to the workflow and don’t feel overwhelmed by new alerts or notifications.
As CDS tools continue to evolve with the growing maturity of machine learning, providers may find the future of clinical decision support to be more intuitive and less intrusive.
At the moment, however, providers should pay close attention to the intersection of workflow and analytics when considering or planning a clinical decision support implementation.