Researchers Use ML to Predict Diet, Chronic Disease Risk
New research from Michigan Medicine shows that machine learning and molecular profiling are reliable tools in predicting patient diet and risk of chronic diseases, like type 2 diabetes.
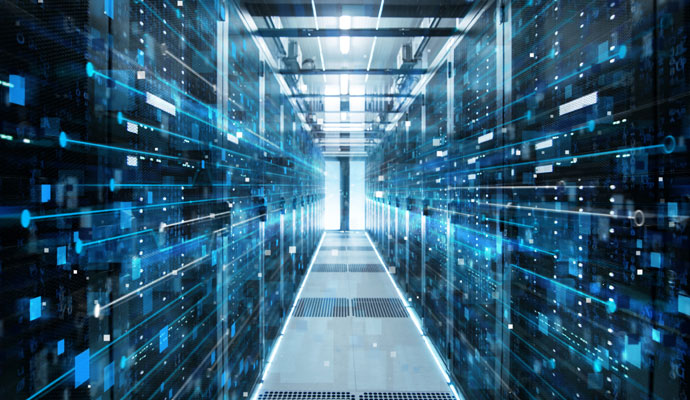
Source: Getty Images
- Led by a Michigan Medicine cardiologist, a recent study found that a novel method using machine learning and molecular profiling can be leveraged to create blood-based dietary signatures that can accurately predict diet and risk of chronic conditions, such as cardiovascular disease and type 2 diabetes.
According to the press release, researchers often find it challenging to know what patients are consuming and, as a result, the health risks associated with their diet.
Traditionally, providers have asked patients to complete food frequency questionnaires to report data such as calorie intake and nutrients. This strategy has limitations, however, which mainly relate to the fact that all reported data is based on patient memory.
In this study, researchers aimed to develop a novel method for accurately predicting patient diet and potential disease risk. This method used molecular profiling and machine learning to create blood-based dietary signatures. To fund the study, researchers received a $170 million grant from the National Institutes of Health, which they gave to clinics nationwide for a Nutrition for Precision Health study that aims to develop algorithms to predict individual responses to food.
“We need tools that are more reliable and precise while also being easy to use for everyone. Using metabolite signatures and data science, we can improve our understanding of how much people are actually taking in, as well as what risks they may incur for cardiometabolic disease that affect millions of Americans,” said senior study author Venkatesh Murthy, MD, PhD, a cardiologist at the University of Michigan Health Frankel Cardiovascular Center and an associate professor of cardiology at U-M Medical School, in the press release.
The study assessed data from over 2,200 White and Black adults, all of whom were participants in the Coronary Artery Risk Development in Young Adults study. Researchers collected blood samples and food surveys to define metabolite signatures of diet as well as the risk of subsequent disease over a 25-year period.
They used a machine-learning model to create a blood-based dietary signature that increased the prediction accuracy of an individual's diet over 19 food groups by about 10 to 20 percent.
Further, the blood-based signature was able to outperform a standard measure of diet quality, called the healthy eating index, in identifying individuals at risk of developing diabetes and cardiovascular disease based on each food group.
“The use of metabolites to understand food exposures and nutrition is an expanding area in nutritional science,” said study co-author Ravi Shah, MD, cardiologist and associate professor of medicine at Vanderbilt University Medical Center, in the press release. “Beyond understanding what types of nutrition are better or worse for our health, the methods here could allow those studying food science to take a metabolic snapshot of nutrition and diet to better understand their implications on health.”
Researchers noted that the blood-based signature technique requires future tests in prospective, controlled studies of several diets.
Similarly, in April, researchers found that using an artificial intelligence (AI)-based system in ultrasound procedures led to a sharp increase in the accuracy of heart disease diagnoses among fetuses. The study noted that the chances of survival increase dramatically when the fetus is diagnosed early. This led researchers to create an AI model to determine a healthy fetal heart.
To do so, they exposed the model to thousands of ultrasound images, defining each as healthy or unhealthy. They then engaged the AI model in another round of deep learning. Additionally, they had experts diagnose the images with and without the assistance of the AI model.
Based on the results, they concluded that the AI-based decision charts led to more accurate heart disease diagnoses.