Researchers to Use Predictive Analytics to Forecast Rare Disease Development
Researchers from University of Florida Health and the University of Pennsylvania will use artificial intelligence-based predictive analytics to identify patients who may develop rare diseases.
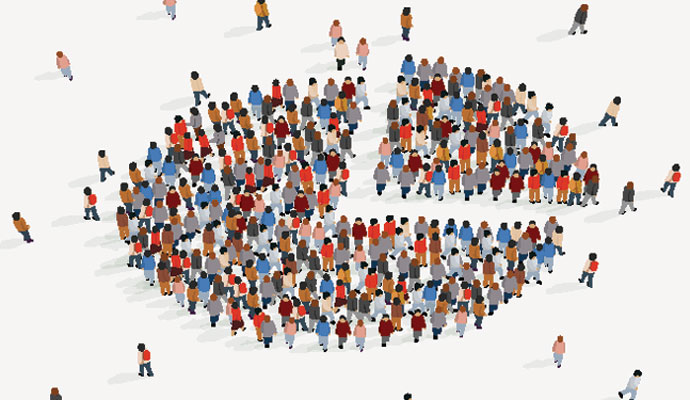
Source: Getty Images
- A research team from University of Florida (UF) Health and the University of Pennsylvania Perelman School of Medicine (Penn Medicine) have announced that they will develop a set of predictive analytics algorithms to forecast which patients appear likely to develop certain rare diseases.
According to the press release, rare diseases can be difficult to diagnose because of their vague or perplexing symptoms and the fact that they affect so few people. Some rare disease patients can go undiagnosed and untreated for years, negatively impacting patient outcomes.
To combat this, the researchers set out to develop artificial intelligence (AI)-based models that can predict which patients may develop one of these diseases. Their method, known as Predictive Analytics via Networked Distributed Algorithms for multi-system diseases (PANDA), is part of this effort.
The project follows a $4.7 million funding award from the National Institutes of Health (NIH). Using the award, the research team will develop a set of machine-learning (ML) algorithms to identify which patients are at risk of five types of vasculitis and two types of spondyloarthritis, including psoriatic arthritis and ankylosing spondylitis, all of which are considered rare diseases in the US.
The press release defines a rare disease as one that affects fewer than 200,000 people nationwide. It states that there are approximately 7,000 of these diseases worldwide, and in the US, about 10 percent of people are affected by a rare disease.
The algorithms will make predictions based on information present in patient EHRs, providing clinical decision support and an increased chance of patients getting diagnosed sooner.
“This is an exciting step forward, building on our current PDA framework, from clinical evidence generation toward AI-informed interventions in clinical decision-making,” said Yong Chen, PhD, a professor of biostatistics at Penn, in the press release. “Despite the clear need to reduce the dangerous and costly delays in diagnosis, individual clinicians, especially in primary care, face important challenges.”
One of these challenges is the lack of access to patient data or information about the patient’s care journey. A lack of familiarity with rare diseases also creates another hurdle. By automatically scanning known patient information to identify rare disease risk, PANDA aims to address these issues.
“The increasing availability of real-world data, such as electronic health records collected through routine care, provides a golden opportunity to generate real-world evidence to inform clinical decision-making,” said Jiang Bian, PhD, a professor at the UF College of Medicine and chief data scientist for UF Health, in the press release. “Nevertheless, to leverage these large collections of real-world data, which are often distributed across multiple sites, novel distributed algorithms like PANDA are much needed.”
Similar efforts to address rare diseases using AI are underway at other institutions.
Last week, researchers from the Mahmood Lab at Harvard Medical School and Brigham and Women’s Hospital shared that a deep-learning algorithm known as Self-Supervised Image Search for Histology (SISH) can act as a search engine for pathology images related to rare diseases. The tool has various applications, but one of the most significant is its potential to support rare disease identification, diagnosis, and treatment.