Omitting Race, Ethnicity from Risk Models May Lead to Health Disparities
New research suggests that using clinical risk prediction models that omit race and ethnicity may worsen predictive accuracy and exacerbate health disparities.
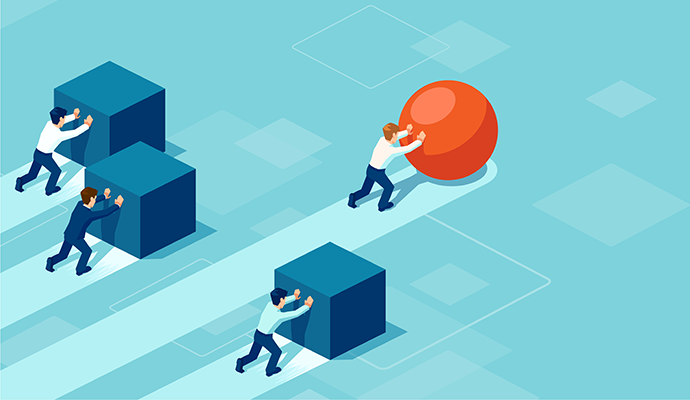
Source: Getty Images
- Researchers have shown that omitting race and ethnicity as predictors in colorectal cancer recurrence risk prediction models may be associated with racial and ethnic biases that contribute to health disparities and worsened patient outcomes, according to a study recently published in JAMA Network Open.
As healthcare systems and providers look toward improving health equity for their patients, some have raised concerns about how the inclusion of race and ethnicity as predictors in clinical risk prediction models may impact outcomes for minoritized patients.
However, the researchers indicated that there is a lack of studies investigating whether omitting race and ethnicity from risk prediction algorithms will impact clinical decision-making for patients in certain racial and ethnic groups.
To help bridge this gap, the researchers evaluated whether including or omitting race and ethnicity as predictive values in a colorectal cancer recurrence risk algorithm may be associated with racial bias. The study defined racial bias as “racial and ethnic differences in model accuracy that could potentially lead to unequal treatment.”
The research team chose to conduct a retrospective prognostic study leveraging EHR and linked cancer registry data from 4,230 Kaiser Permanente Southern California (KPSC) patients with colorectal cancer who received primary treatment between 2008 and 2013 and follow-up until December 31, 2018.
Four prediction models were tasked with predicting time from surveillance start to cancer recurrence: a ‘race-neutral’ model that explicitly excluded race and ethnicity as predictor values, a ‘race-sensitive’ model that included race and ethnicity, a model that included two-way interactions between clinical predictors and race and ethnicity, and separate models by race and ethnicity.
The algorithmic fairness of each model was then measured using false-positive and false-negative rates, positive predictive value (PPV), negative predictive value (NPV), model calibration, and discriminative ability.
The researchers found that the ‘race-neutral’ model demonstrated poorer performance in terms of false-negative rates, NPV, and calibration among racial and ethnic minority subgroups compared to non-Hispanic white individuals.
Overall, adding race and ethnicity as predictive values improved algorithmic fairness in false negative rates, PPV, calibration slope, and discriminative ability. Models that included race interaction considerations or were stratified by race did not improve model fairness, which the researchers indicated may be a result of small subgroup sample sizes.
These findings show that removing race and ethnicity from clinical risk prediction models may worsen algorithmic fairness, which could contribute to inappropriate care recommendations and health disparities for minoritized patients.
The researchers recommended that clinical model development should include assessments of fairness criteria to help gain insights into how removing factors like race and ethnicity may impact health inequities.
This research comes as healthcare providers grow increasingly concerned about the potential for bias in risk prediction models.
In May, researchers determined that artificial intelligence (AI) models for type 2 diabetes, typically used for screening and prediction, may contain biases that can contribute to over- or under-estimations of a patient's risk based on their race.
The study looked at three models to evaluate whether they show racial bias between non-Hispanic Black and non-Hispanic white populations: the Prediabetes Risk Test (PRT), the Framingham Offspring Risk Score, and the ARIC Model.
All three were found to be miscalibrated in terms of race, over- and under-estimating risk for certain subgroups, which the research team indicated could lead to over- or under-treatment.