Machine learning predicts risk of suicide in patients initiating care
A machine learning tool to predict suicide attempts can accurately stratify high-risk patients scheduled for outpatient mental healthcare intake appointments.
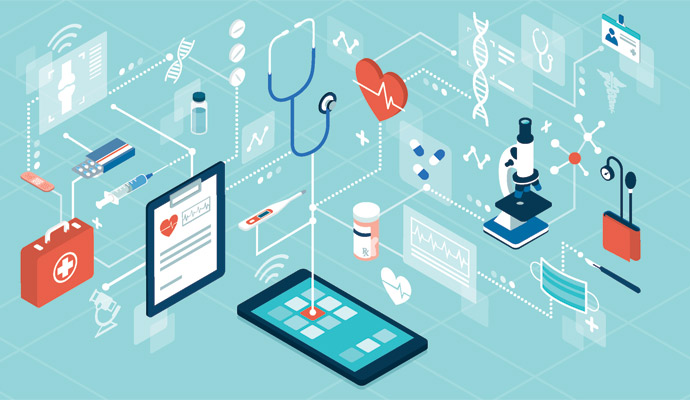
Source: Getty Images
- Kaiser Permanente researchers have demonstrated that a machine learning-based predictive model can stratify suicide risk among patients scheduled for an intake visit to outpatient mental healthcare, according to a study published recently in JAMA Psychiatry.
The research team emphasized that identifying individuals at risk for a suicide attempt early on in their care is crucial, as many patients stop mental health treatment after only one or two visits. However, doing so presents a challenge in the case of patients newly seeking treatment, as their records often lack an extensive mental health history.
Alongside this lack of data, increasing suicide rates and demand for mental healthcare in the United States further underscore the immediate need for accurate clinical risk tools to improve patient outcomes.
The researchers indicated that suicide risk prediction models have already been developed using outpatient mental health encounters, but the performance of these models using intake encounters has yet to be thoroughly assessed.
To address this, the research team evaluated the performance of a previously validated predictive model when tasked with stratifying risk among a cohort of individuals scheduled for an intake visit.
The model is designed to forecast the potential for self-harm and suicide in the 90 days following a mental health encounter.
“This model has already been developed and validated, and now the research is focusing on what we can do with this powerful tool,” said senior author Esti Iturralde, PhD, a research scientist at Kaiser Permanente’s Division of Research (DOR), in a news release. “This study takes us another step forward in understanding how the predictive model would work with a particular group of patients — those seeking mental health care for the first time, rather than those in ongoing care.”
The model was fed data from the 1,623,232 scheduled mental health intake appointments of 835,616 patients seen from January 2012 to April 2022 at Kaiser Permanente Northern California (KPNC). These appointments were either first-time mental health visits or the first visit after a gap of two years or more since the patient’s last encounter.
Using these data, the research team tallied all suicide attempts recorded in the medical record in the 90 days after the scheduled appointment: approximately 0.17 percent of appointments were followed by a suicide attempt.
This information also allowed the model to accurately classify patients based on their suicide risk. The ten percent of appointments flagged as highest risk comprised 48.8 percent of the appointments followed by a suicide attempt.
The tool had an area under the receiver operating characteristic curve of 0.77, an area under the precision-recall curve of 0.02, an expected calibration error of 0.0012 and a sensitivity of 37.2 percent at 95 percent specificity.
These findings led the researchers to conclude that information from mental healthcare intake appointments could be used to accurately stratify suicide risk and guide preventive interventions.
“This study suggests that applying the model at this early point in a patient’s journey can give us useful information,” noted co-author Honor Hsin, MD, PhD, a psychiatrist with The Permanente Medical Group. “This analysis was a necessary step and serves as a foundation for determining if we could use it in the real world.”
“The benefit of a model like this is it pulls from past information about a patient, such as previous medications or past diagnoses,” Hsin continued. “It may provide more context about the patient or reveal risk that is not evident through the other tools clinicians use.”
Despite the model’s success, the authors cautioned that it can only augment, rather than replace, the expertise of clinicians.
Some health systems have already seen success following the deployment of suicide risk stratification tools.
In a recent interview on Healthcare Strategies, leaders from Parkland Health & Hospital System (PHHS) and Parkland Center for Clinical Innovation (PCCI) in Dallas, Texas detailed how the health system’s Universal Suicide Screening Program has had a significant positive impact on suicide prevention efforts.