Machine Learning Identifies Genetic Mutations for Spinal Disorder
To treat and prevent spina bifida, researchers are using machine learning to examine genetic mutations associated with the condition.
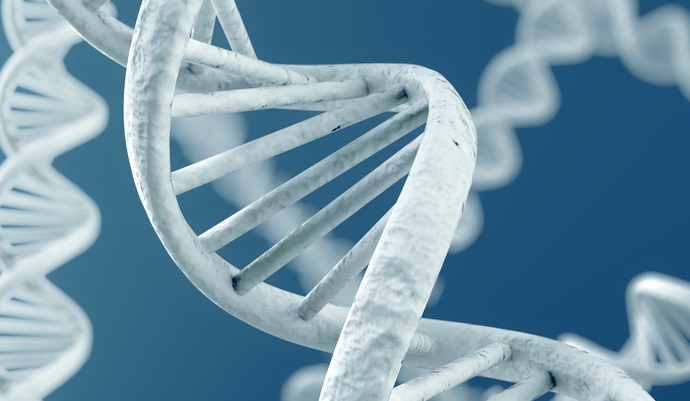
Source: Getty Images
- Weill Cornell Medicine researchers are using machine learning to study genetic mutations associated with spina bifida.
According to senior author and director of the Center for Neurogenetics, Margaret Elizabeth Ross, MD, PhD, the study “brings us closer to being able to provide a precision medicine approach to families who are looking to ensure healthy birth outcomes and the greatest potential for infants affected by spina bifida,” Ross said in a press release.
Spina bifida is a complex genetic disorder, meaning it’s caused by several gene alternations rather than just one. Environmental conditions, including nutrition, medication, and supplements, can impact fetal health.
“The challenge is to understand the role of genetic variation in individual families, coupled with the environmental factors, so we can do our best to ensure a healthy baby,” said Ross, who also heads the Laboratory of Neurogenetics and Development at Weill Cornell Medicine.
To find ways to treat and prevent spina bifida, researchers must reduce bias in patient selection. By collaborating with Baylor College of Medicine and Stanford Medicine, Well Cornell Medicine researchers developed an unbiased approach to study a smaller sample of people and identify genes that distinguish patients with spina bifida versus individuals without the condition.
The research team examined the genomes of 149 people with spina bifida and 149 health controls with similar genetic backgrounds. With machine learning, the team determined which genes with predicted function-changing variants have the greatest potential for differentiating cases from controls.
The research team then studied how these genes related to different activities at the molecular level. The most significant pathways involved glucose and lipid metabolism.
“These processes are relevant to conditions like diabetes and obesity. This really gave us a lot of encouragement that our machine learning approach was coming up with clinically relevant information,” Ross said.
“We continue to build an international consortium of clinicians and families to increase the power of this approach toward understanding human spina bifida.”
Using the machine learning approach, Ross hopes it will be possible to examine genomes for couples who want to conceive, identifying the best approaches to prevent spina bifida. For example, additional folic acid can be an effective preventive measure, while for others, taking supplements like inositol can decrease risk.
“One day we will be able to counsel individual couples on what is the most effective route for them to have a healthy birth outcome, and for a child affected by spina bifida, to optimize their development and quality of life into adulthood,” Ross said.