Machine-Learning Approach Aims to Optimize Rare Disease Diagnosis
New research from Stanford University describes the creation of a machine-learning framework that could predict a comprehensive set of diagnosis codes for rare disease patients.
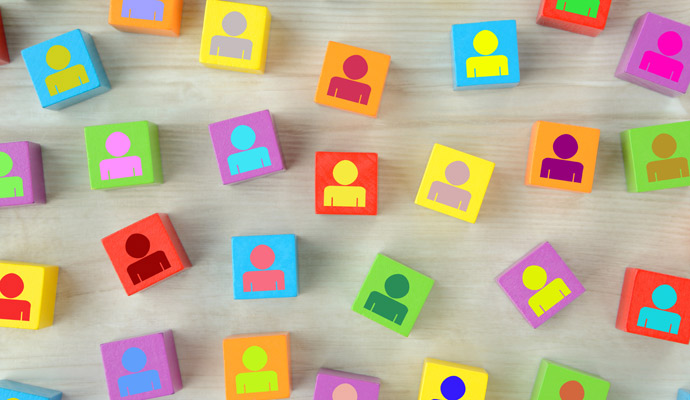
Source: Getty Images
- A recent study from Stanford University Human-Centered Artificial Intelligence (Stanford HAI) described the creation of a machine-learning framework, known as POPDx, that aims to enhance the rare disease detection process by predicting a comprehensive set of diagnosis codes.
The framework was created to predict diagnosis codes for all patients in the UK Biobank. Biobanks can provide researchers with valuable information as they make connections between external contributions and disease trends. Biobanks are datasets that contain genetic and health information which can provide important insights for those who use them.
However, difficulties such as information gaps as well as limited quantity and quality may contribute to research barriers. This led a group of Stanford HAI researchers to develop a novel strategy to predict diagnosis codes.
Known as POPDx, the model aims to predict a comprehensive set of diagnosis codes for the half a million participants in the UK Biobank, including patients with rare diseases. It assesses relationships between patient data and disease information and leverages natural language processing and the Human Disease Ontology to make probabilistic decisions, according to the press release. The Human Disease Ontology provides descriptions of human disease terms, phenotype characteristics, and related medical vocabulary.
Researchers noted that POPDx could outperform traditional models as it can predict diseases that do not exist in the training data.
“While most machine learning approaches that use deep neural networks require a ton of training, we were very pleased that our approach using prior knowledge like text and taxonomy allowed us to recognize some diseases in our test set, even though we had never seen them before in training. This is important because while there is substantial data in medicine, it is not at the same scale as large IT companies, and so it is critical that we develop methods that can work on sparse data, and work well enough to help patients with uncommon diseases,” said Russ Altman, MD, PhD, a Stanford HAI associate director and professor of bioengineering, genetics, medicine, biomedical data science, and computer science, in a press release.
Researchers also noted that POPDx uses a broad range of data. The model examines demographics, patient questionnaires, medical exams, EHRs, physical data, and lab tests.
“Before this, most of the existing models needed well-curated datasets, which means they might not be able to look into the abundance of features that we are able to look into with our work,” said Lu Yang, a Stanford PhD student, in the press release. “Usually research will be specific to a certain domain, like heart disease, so they’ll only look at that relevant information or codes. But for our study we tried to come up with a complete profile of the UK Biobank participants.”
According to the press release, Yang improved the model's areas under precision-recall curves (AUPRC) — a common metric for evaluating classification performance for imbalanced datasets — by 218 percent for unseen diseases and 151 percent for rare diseases. Thus, unlike typical machine-learning models that require large datasets, POPDx displayed high performance with limited data.
There were, however, difficulties associated with the development of POPDx. For instance, the demographics of the study population lacked diversity, which could have led to bias. But the team used background information on the hierarchy and relationship between diseases to mitigate this issue, the press release noted.
Machine-learning use in clinical care is growing as research highlights its efficacy.
A study from December 2022 described how using preoperative data and intraoperative hemodynamic monitoring data within a machine-learning prediction model led to accurate predictions for massive transfusion needs, allowing for early intervention for high-risk patients.
Another machine-learning algorithm created in February by researchers from Dell Children’s Medical Center of Central Texas can track infants' movement patterns in the neonatal intensive care unit, which could indicate medical risks.