Gun Violence Archive Data May Bolster Community Firearm Violence Research
New research shows that independently collected gun violence data may provide unique advantages for researchers studying community firearm violence in large cities.
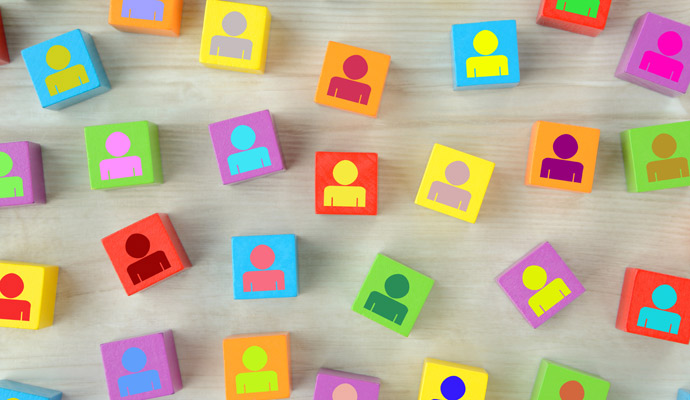
Source: Getty Images
- A study published recently in JAMA Network Open shows that the Gun Violence Archive (GVA), an independent data collection group, may be a limited, but valid source of data for researchers investigating community firearm violence in large cities.
The research team explained that firearm injury is a significant public health concern in the United States, but that no single, validated source of national data currently exists to study the phenomenon.
The GVA has generated a firearm violence event dataset that uses public records and media, in addition to location information similar to that leveraged by police departments, to provide insights into gun violence across the US.
The researchers indicated that the GVA has the potential to expand the scope, timeliness, and flexibility of firearm injury research, but experts have questioned the validity of GVA’s data collection methods.
To assess the GVA’s potential as an epidemiologic data source for community firearm violence, including firearm homicide and nonfatal shootings stemming from interpersonal violence, the research team undertook a cross-sectional observational study.
The study compared community firearm violence data from the GVA to publicly available police department data, which are the standard data used in this type of research. Data were collected between January 1, 2015, and December 31, 2020 in four cities with populations greater than 300,000 people per 2020 US Census information: Philadelphia, Pennsylvania; New York, New York; Chicago, Illinois; and Cincinnati, Ohio.
From there, community firearm violence events from the GVA were matched to police department shooting events based on location and date. For each dataset, the researchers calculated the sensitivity and positive predictive value to help evaluate performance.
During the study period, the GVA documented 26,679 shooting events, while police department data documented 32,588.
A total of 29,791 individuals were involved in shootings reported in both datasets. Of these, 87.3 percent were involved in shootings with multiple injured individuals, and 69.7 percent were involved in nonfatal shootings.
The researchers found that the GVA was missing 9,643 individuals who were involved in shootings, of which 82.1 percent involved single injured individuals and 62.6 percent were nonfatal. Shootings that involved women or multiple individuals were less likely to be missing from the GVA database, which the research team posited suggests a “systematic missingness” in the data.
Overall, the sensitivity of the GVA was found to be 81.1, and sensitivity was shown to steadily improve over the six-year study period. The dataset’s positive predictive value was 99.0 percent.
The researchers concluded that these findings support the use of the GVA in certain research, including studies that require features unique to the GVA, such as timeliness, geographic coverage, and spatial resolution. However, they cautioned that the GVA’s bias toward shootings involving women, children, and multiple individuals limits its use for more granular examinations of epidemiological trends in this area.
Data-driven studies of firearm violence are one strategy healthcare stakeholders have undertaken to combat US gun violence, with some health systems investigating the potential for gun safety conversations in patient-provider communication as concerns about crime and violence as social determinants of health (SDOH) grow.
Researchers are also examining how artificial intelligence (AI) could help inform firearm violence prevention strategies.
Last year, a research team demonstrated that machine learning (ML) models trained using administrative data on handgun transactions can accurately predict firearm suicide risk.
This information could lead to insights to better inform targeted suicide prevention interventions.
Using such an ML model resulted in moderately informative predictions, and the analysis identified fifteen variables most important for firearm suicide risk forecasting, including purchaser age, race, and ethnicity, in addition to factors like handgun category.