Google Deep Learning Model Detects Tuberculosis On Par With Radiologists
Google has developed a deep-learning model that can detect tuberculosis as accurately as radiologists using chest radiographs.
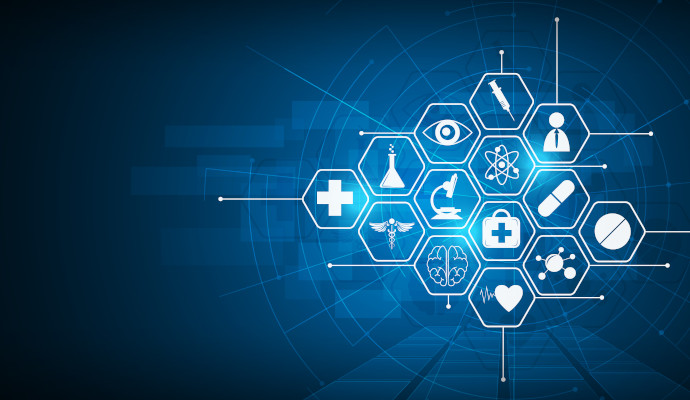
Source: Getty Images
- New research published this week in the journal Radiology found that a deep-learning (DL) system developed by Google performs on par with radiologists at detecting active pulmonary tuberculosis (TB) using chest radiographs.
According to the World Health Organization (WHO), TB is a bacterial infection that usually affects the lungs and spreads when an infected individual coughs, sneezes, or spits. The disease creates a significant global health burden, with 10 million people falling ill each year worldwide. Of these, 1.5 million people die annually, despite TB being preventable and curable.
WHO further notes that the disease is one of the world's top killers in terms of infectious disease, and it is a leading cause of death for those with HIV. It is estimated that a quarter of the global population is infected with TB bacteria, but most people who fall ill with TB live in low- and middle-income countries.
This global disease burden has led to significant efforts to detect and eliminate TB, but numerous challenges have limited progress. As part of its strategy to facilitate early detection of TB, the WHO recommends chest radiographs to facilitate TB screening. However, the study authors note that the lack of chest radiograph interpretation expertise in many regions hampers these efforts.
To address this, the researchers sought to develop a DL model capable of detecting active pulmonary TB. The model was trained and tested using a retrospective dataset of chest radiographs acquired from 1996 to 2020. The data were sourced from patients in 10 countries with positive TB results confirmed using microbiological tests or nucleic acid amplification testing (NAAT).
The model was evaluated using a four-country test set containing data from China, India, the US, and Zambia, and a dataset containing radiographs from a mining population in South Africa. The model’s performance was then compared with that of 14 radiologists. The model was designed to achieve targets of 90 percent sensitivity or 70 percent specificity, in line with WHO guidelines for this type of technology.
Overall, the model performed on par with the radiologists. The model achieved a sensitivity of 88 percent compared to 75 percent for radiologists. But it achieved a specificity of 79 percent compared to 84 percent for radiologists.
These findings indicate that the model may be able to facilitate TB screening in areas with limited radiologist resources, the researchers concluded. However, more research and clinical validation of the model are needed before it can be integrated into clinical settings.
This research is the latest in a growing effort to use artificial intelligence (AI) to enhance medical imaging.
This week, researchers found that machine-learning (ML) models developed using a crowdsourcing approach can improve efforts to quantify radiographic joint damage in patients with rheumatoid arthritis.
Last week, a three-part special report outlined best practices for mitigating bias in AI- and ML-based radiology tools.