Genomic Analyses Predict Therapy Response in Lung Cancer Patients
Multimodal genomic analyses could help physicians predict which patients with lung cancer will respond to immunotherapy.
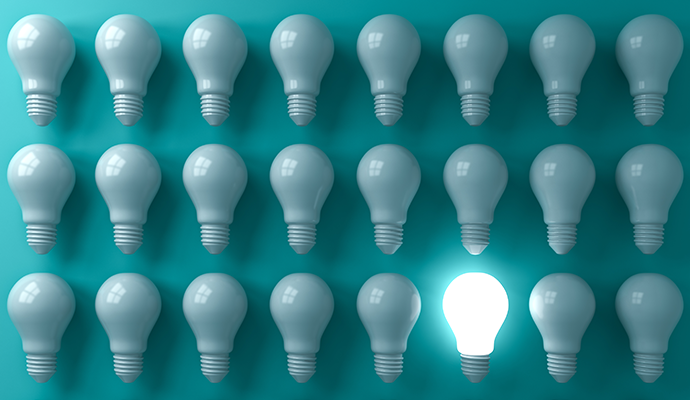
Source: Getty Images
- An integrated genomic approach could help providers predict which patients with nonsmall cell lung cancer will respond to therapy with immune checkpoint inhibitors.
Immune checkpoint inhibitors are changing the field of cancer therapeutics, but there is a lack of biomarkers that identify which patients are most likely to respond to this type of treatment.
Tumor mutational burden (TMB), a measure of the number of mutations carried by tumor cells, is an emerging biomarker of response. However, TMB values are confounded by the tumor purity, or the amount of tumor versus normal cells, of the sample analyzed.
Researchers from Johns Hopkins Kimmel Cancer Center, the Bloomberg-Kimmel Institute for Cancer Immunotherapy, and the Johns Hopkins University School of Medicine, developed a computational approach that more accurately computes TMB.
The team also developed an integrated model of response that combined corrected TMB with nuanced genomic features and each patient’s antigen presentation ability. Providers could also use the method to accurately estimate TMB and optimize prediction of response to immunotherapy among patients with lung cancer, colon cancer, melanoma, and other solid tumors.
READ MORE: Evaluating the Benefits and Challenges of Genomics in Healthcare
“Immunotherapy is an exciting treatment modality for many tumors, but what we don’t truly know is who will respond to immunotherapy and why, and if there are specific molecular features that can help predict response,” said Valsamo Anagnostou, MD, PhD.
Current biomarkers used to evaluate patients’ response to immunotherapy include a test to measure the amount of the protein PD-L1 on cancer cells and TMB.
“There are more and more studies coming out that show TMB is actually not as predictive as we thought it would be,” said Victor Velculescu, MD, PhD, professor of oncology and the study’s senior author.
“Some tumors with a high TMB do not respond to immunotherapy, and some tumors with low TMB benefit from immunotherapy. There is an urgent need to develop integrated biomarkers that explain the nuances of the tumor-immune system crosstalk that can better inform us in terms of the clinical course of the patient.”
The team evaluated 3,788 tumor samples from the National Cancer Institute’s Cancer Genome Atlas database, as well as 1,661 tumor samples from a previously published cohort of patients treated with immunotherapy. Researchers investigated the complexities of observed TMB estimates from whole exome sequencing and targeted next-generation sequencing.
The group found a significant correlation between TMB and tumor purity, meaning the higher the tumor purity, the closer it is to the true TMB of the tumor. The lower the tumor purity, the more likely the TMB estimate will be inaccurate.
“Observed TMB is strongly affected by low tumor purity, and this simple concept is completely underestimated in the clinical setting,” said Noushin Niknafs, PhD, postdoctoral fellow and co-first author of the study.
Researchers developed a computational approach to estimate corrected TMB values for each tumor based on tumor purity. They simulated 20,000 tumors with various levels of TMB and sequencing coverage and generated a correction factor for each simulated tumor based on its purity.
“The correction factors can be summarized in a user-friendly look up table,” said Anagnostou. “For example, if a tumor sample had a purity of 20 percent to 30 percent, a clinician could look at the table and see a coefficient to multiply the sample by to better achieve true TMB.”
Researchers also developed an approach to correct TMB derived from targeted sequence data. In a reanalysis of 1,661 tumor samples treated with immune checkpoint inhibitors, the team found that using corrected TMB estimates improved prediction of overall survival.
The group also wanted to understand additional molecular features that play a part in patient response to immunotherapy. They performed whole exome sequencing for 104 lung tumors treated with immune checkpoint inhibitors.
Through comprehensive analyses of sequence and structural alterations, they found more activating mutations in receptor tyrosine kinase (RTK) genes – receptors that are key regulators of cell processes including cell proliferation, survival, and metabolism – among tumors that did not respond to immunotherapy in several cohorts of patients.
Researchers also identified a predominance of smoking related mutations in patients that respond to therapy. Together, corrected TMB, RTK mutations, the mutation smoking signature, and the number of germline variants of human leukocyte antigen (HLA) provided the team with a much more accurate prediction of which patients would respond to immunotherapy compared to TMB alone.
“We expect this approach is going to be incorporated into clinical practice, and it can change the way providers make decisions about their patients,” Anagnostou said.
“For example, if a clinician can know with certainty that the tumor has a high tumor mutation burden, they may choose to give immunotherapy as stand-alone therapy, whereas if the tumor has a low tumor mutation burden, they may choose to give chemotherapy plus immunotherapy.”