Deep-Learning Tool May Facilitate Monkeypox, Skin Disease Identification
A mobile application that uses deep learning to analyze skin lesion images can detect monkeypox with 91 percent accuracy and could be trained to identify the presence of other skin diseases.
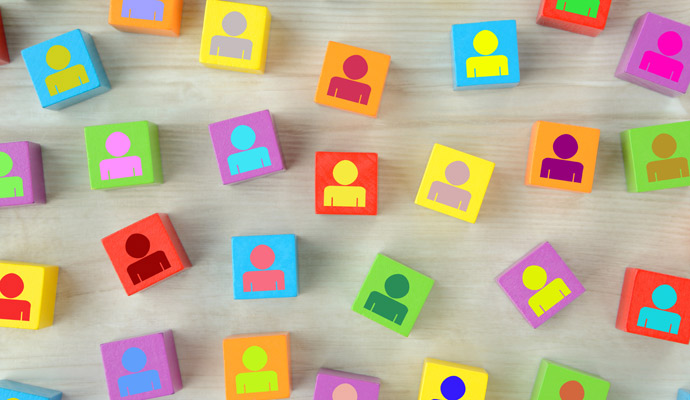
Source: Getty Images
- A study published this month in the Journal of Medical Systems shows that a deep learning (DL)-based mobile application can accurately identify monkeypox infection using images of skin lesions.
On July 23, the World Health Organization (WHO) declared the 2022 global monkeypox outbreak a Public Health Emergency of International Concern (PHEIC) following reported cases of the disease in 75 countries. Shortly afterward, in August, the US Department of Health and Human Services (HHS) declared the ongoing spread of the disease across the country a public health emergency (PHE).
Monkeypox is a smallpox-like disease characterized by fever, chills, headache, swelling of the lymph nodes, muscle aches, fatigue, respiratory symptoms, and painful skin eruptions, according to the Centers for Disease Control and Prevention (CDC). People with monkeypox also get a rash that can initially appear to be painful or itchy pimples and blisters but will progress through several stages, including scabs, before finally healing.
Part of the CDC’s monkeypox monitoring efforts is encouraging people with new or unexplained rashes to avoid contact with others and see a healthcare provider, who can then examine the skin lesions and determine if monkeypox infection is present. However, healthcare access and efficiency issues can impact how quickly patients are screened and diagnosed, which can influence the rate of monkeypox spread.
To combat this, the researchers who published the study sought to develop a preliminary diagnostic tool that could accurately identify monkeypox and encourage infected individuals to see a provider and isolate themselves more quickly.
The research team created an Android mobile application that uses artificial intelligence (AI) to analyze images of skin lesions taken using the mobile device’s camera.
The researchers leveraged a deep convolutional neural network (CNN) for this task. CNNs, a type of DL, are often used to understand visual data as they can analyze images and extract features that can be used to classify the images into categories. These networks are often implemented in medical imaging because their ability to classify images can be used to diagnose various conditions.
In this case, the research team gathered and prepared human monkeypox lesion images using image preprocessing methods. The researchers then leveraged transfer learning and other methods to train their network using high-performing pre-trained networks and the lesion image dataset.
Their model, known as TensorFlow, was converted into the TensorFlow Lite model for use on mobile devices. Following this, they developed an Android mobile application incorporating the TensorFlow Lite model.
When the mobile application is launched, it automatically opens the device’s camera and takes a real-time video stream. Images from this video stream are then fed to the model’s CNN for analysis. From there, the tool extracts features from the image, classifies them, and indicates to the user whether the classification is positive or negative for monkeypox.
When testing the tool, the researchers deployed the mobile application on three separate Android devices and measured its accuracy and the time it took for the tool to generate inferences. Overall, the tool achieved high performance across devices, with average inference times of 197, 91, and 138 milliseconds. The system was also capable of classifying the images with 91.11 percent accuracy.
These findings indicate that such a tool could allow users who suspect they have a monkeypox infection to conduct preliminary screenings from home and enable them to take action in the early stages of infection, the researchers stated. This, in turn, may help reduce the spread rate of the disease.
The research team further concluded that the proposed tool could be expanded to classify different skin diseases outside of monkeypox.