Deep Learning Model Flags Non-Smokers at Increased Risk for Lung Cancer
A new model has shown that among non-smokers, there is a 2.1 times greater risk of developing lung cancer for those in high-risk groups compared to their peers.
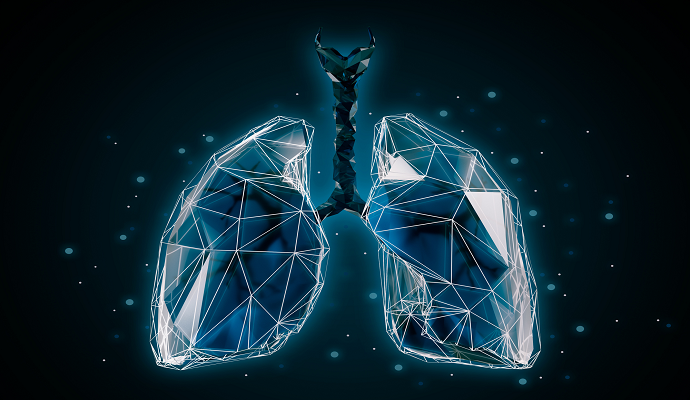
Source: Getty Images
- A deep learning model can accurately identify non-smokers at high risk for lung cancer using only routine chest X-rays, according to research presented at this year’s meeting of the Radiological Society of North America (RSNA).
The Centers for Disease Control and Prevention (CDC) indicate that lung cancer is the third most common cancer in the United States, and more Americans die from the disease than any other type of cancer.
The American Cancer Society reports that smoking is a leading cause of lung cancer, with approximately 80 percent of lung cancer deaths estimated to result from smoking. This rate is believed to be even higher for certain types of lung cancer, such as small cell lung cancer (SCLC).
However, the researchers noted that roughly ten to 20 percent of lung cancers occur in “never-smokers,” individuals who have never smoked or have smoked less than one hundred cigarettes in their lifetime. They further noted that lung cancer among never-smokers is on the rise, and these cancers are often more advanced than those found in non-smokers when they are discovered.
These trends highlight the importance of screening, but current guidance from the United States Preventive Services Task Force (USPSTF) does not recommend lung cancer screening for never-smokers.
READ MORE: Risk-Based Lung Cancer Screening May Reduce Racial, Ethnic Disparities
“Current Medicare and USPSTF guidelines recommend lung cancer screening CT only for individuals with a substantial smoking history,” said the study’s lead author, Anika S. Walia, a medical student at Boston University School of Medicine and researcher at the Cardiovascular Imaging Research Center (CIRC) at Massachusetts General Hospital (MGH) and Harvard Medical School in Boston, in the press release. “However, lung cancer is increasingly common in never-smokers and often presents at an advanced stage.”
The USPSTF currently recommends that adults between the ages of 50 and 80 who have at least a 20 pack-year smoking history and currently smoke or have quit within the past 15 years undergo annual lung cancer screening via low-dose computed tomography (LDCT).
Screening is not recommended for never-smokers because the potential harms of screening outweigh the benefits. Further, the research team indicated that predicting lung cancer in this population is a challenge, as existing methods to determine risk require information or test results not readily available to most patients, like family history of the disease, serum biomarkers, and pulmonary function testing.
To address this, the researchers sought to develop an artificial intelligence (AI) model that could identify lung cancer risk in never-smokers using routine clinical data.
The resulting tool, a deep learning model known as CXR-Lung-Risk, is a risk prediction model designed to leverage chest X-ray images from the electronic health record (EHR). The model searches these images for patterns associated with lung cancer to stratify patients into risk groups.
READ MORE: Blood Test-Based Model Accurately Predicts Lung Cancer Death Risk
“A major advantage to our approach is that it only requires a single chest-X-ray image, which is one of the most common tests in medicine and widely available in the electronic medical record,” Walia explained.
The tool was developed using 147,497 chest X-rays of 40,643 asymptomatic smokers and never-smokers from the Prostate, Lung, Colorectal, and Ovarian (PLCO) cancer screening trial. The model was then externally validated on a separate cohort of never-smokers who received outpatient chest X-rays from 2013 to 2014.
Using these data, six-year incident lung cancer was then identified using International Classification of Disease codes. From there, risk scores generated by the model were converted into low-, moderate-, and high-risk groups based on externally derived risk thresholds.
Of the 17,407 study participants, 28 percent were flagged as high risk by the deep learning tool, and 2.9 percent of these patients were later found to have lung cancer. The research team underscored that these numbers are beyond the 1.3 percent six-year risk threshold where lung cancer screening is recommended by the National Comprehensive Cancer Network.
Following adjustments for participants’ age, sex, race, previous lower respiratory tract infection, and prevalent chronic obstructive pulmonary disease, the researchers found that non-smokers in the high-risk group were at 2.1 times greater risk of developing lung cancer than their low-risk group counterparts.
READ MORE: University of Houston AI Project Aims to Improve Lung Cancer Diagnostics
“This AI tool opens the door for opportunistic screening for never-smokers at high risk of lung cancer, using existing chest X-rays in the electronic medical record,” said senior author Michael T. Lu, MD, MPH, director of artificial intelligence and co-director of CIRC at MGH. “Since cigarette smoking rates are declining, approaches to detect lung cancer early in those who do not smoke are going to be increasingly important.”
Deep learning has increasingly demonstrated its potential to help identify patients who may benefit from lung cancer screening.
In January, researchers found that one such model was able to predict lung cancer risk using chest X-rays and other EHR data while also flagging individuals on Medicare who are ineligible, but may benefit from, lung cancer screening.
US Centers for Medicare & Medicaid Services (CMS) coverage enables screening for individuals aged 55 to 77 years with a smoking history of 30 pack-years or more who are currently smoking cigarettes or quit within the past 15 years.
However, a significant number of cancers occur in those ineligible under CMS guidelines. Recent expansions to eligibility have sought to address this, but these did not tackle the issue of poor participation in lung cancer screening.
To overcome these challenges, the research team built an automated lung cancer risk tool to complement CMS criteria.