Coalition for Health AI Unveils Draft Blueprint for Ethical AI Implementation
In a new draft report, the Coalition for Health AI makes recommendations for guidance, guardrails, best practices, and governance to help ensure ethical healthcare AI implementation.
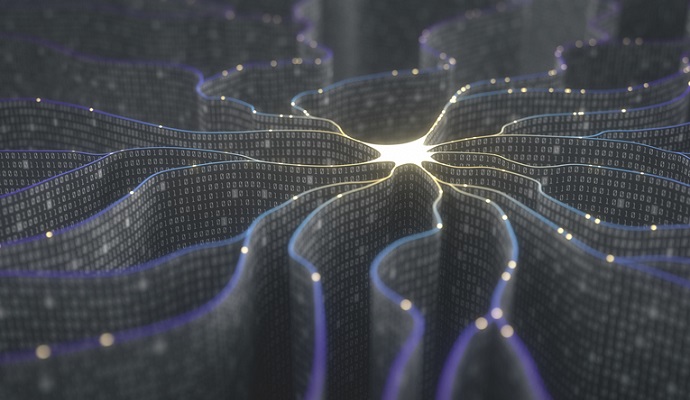
Source: Thinkstock
- The Coalition for Health AI (CHAI) has published a draft of its ‘Blueprint for Trustworthy AI Implementation Guidance and Assurance for Healthcare,’ which outlines recommendations for ethical health artificial intelligence (AI) guidelines to support high-quality care and increase AI credibility.
CHAI launched in March as an initiative for health systems, organizations, AI and data science experts, and other healthcare stakeholders to advance health AI while simultaneously addressing health equity and bias. To work toward these goals, the coalition aims to identify where standards, best practices, and guidance need to be developed for AI-related research, technology, and policy.
The blueprint states that health AI offers significant potential for advancing medical research and improving clinical care. But the technology currently has minimal applications fit for clinical use because AI tools can perpetuate biases and increase harmful outcomes if they are not developed with health impact, fairness, and equity across all populations in mind.
The report further notes that the lack of consensus-based standards or guidance surrounding healthcare AI can, and in some cases, has led to multiple approaches preventing developers and other stakeholders from knowing what standards to adopt and how. The overwhelming amount of potentially conflicting or discordant approaches can lead to distrust of AI.
The blueprint aims to provide a potential, consensus-based framework to help address these issues and guide ethical health AI implementation. The report outlines several elements that must be addressed to ensure trustworthy AI use in healthcare: bias, equity, fairness, testability, usability, safety, transparency, reliability, and monitoring.
CHAI defines bias as “disparate performance or outcomes for selected groups defined by protected attributes such as race and ethnicity, and in this paper, differences that are perpetuated and/or exacerbated by AI models and their use.” Under this framework, bias, equity, and fairness are interrelated. Addressing issues in these areas requires leveraging health equity by design in all steps of AI policy, regulation, development, evaluation, and validation processes.
Testability helps ensure a strong understanding of the model and its intended use, including where, why, and how it is used, and whether its performance can be verified as satisfactory within that context. Usability considerations take into account the quality of the user’s experience, including effectiveness, efficiency, and satisfaction when using an algorithm’s output.
Safety aims to prevent adverse outcomes from a model’s use, and transparency measures an algorithm’s interoperability, traceability, and explainability. Reliability measures an AI’s ability to perform its required function under certain conditions, while monitoring is concerned with ongoing surveillance of a model to look for and flag failures and vulnerabilities to minimize potential adverse effects.
CHAI also outlines three steps to employ AI tools in a way that benefits patients, is equitable, and promotes the ethical use of AI.
The first is setting up an assurance accreditation lab and associated technical assistance service, which may help achieve results through health system preparation, AI tool use, and development of an infrastructure for enabling trustworthy AI, the report states. Such a lab would define value and associated infrastructure components, including registries for tools and legal agreements for testing tools on relevant data.
The second step is concerned with institutionalizing trustworthy AI systems within healthcare organizations. This process is designed to establish organizational structures, oversight processes, and evaluation metrics against which to measure health systems working toward enabling ethical AI.
The final step describes “energizing a coalition of the willing.” In this step, CHAI discusses the need for collaboration to help identify priorities, catalyze action, create incentives, and engage and educate the stakeholder community around a national framework for trustworthy health AI.
The report's authors conclude that this convening of stakeholders will help move the field of ethical healthcare AI forward and foster a community for implementation and adoption.
CHAI’s blueprint is the culmination of multiple meetings and discussions this past year among academic, industry, and government experts in healthcare and AI, including leadership from Johns Hopkins University, Mayo Clinic, Google, Microsoft, and Stanford Medicine, in addition to US federal observers from the US Food and Drug Administration (FDA), the Office of the National Coordinator in Health Information Technology (ONC), the National Institutes of Health (NIH), and the White House Office of Science and Technology Policy (OSTP).