Atlas Resource Aims to Support Custom Kidney Disease Treatment
Researchers created a new atlas tool that analyzes various cell types and states to determine causes and treatment paths for kidney disease.
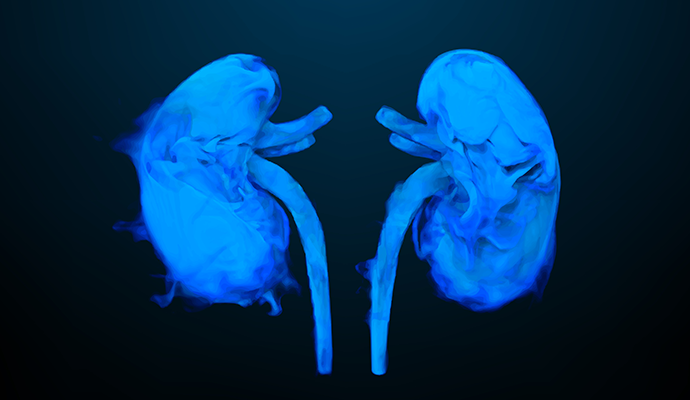
Source: Getty Images
- Supported by funding from the National Institutes of Health (NIH), a collaborative effort between UC San Diego, Washington University, and other organizations led to the creation of an atlas tool designed to promote treatment for kidney disease through cell and nuclei analysis.
Affecting millions of Americans, kidney disease is a serious condition that can be hard to navigate and treat. Chronic kidney disease (CKD) is especially common, impacting 35.5 million US adults, according to the Centers for Disease Control and Prevention.
Given the complexity of the organ, treatment for kidney conditions can be difficult to assess and study. The new Kidney Tissue Atlas, however, takes a new approach.
Researchers constructed the Kidney Tissue Atlas by analyzing over 400,000 cells and nuclei from healthy and unhealthy kidneys. This tool uses maps of 51 main kidney cell types, encompassing rare and newer ones. It also consists of 28 cellular states that represent illness or injury, along with a repository of raw gene data, models of cells, and microenvironment relationships from 45 healthy donor kidneys and 48 kidney disease biopsies. This atlas is part of the Kidney Precision Medicine Project (KPMP).
“We want to understand that progression at the single-cell level,” said study co-first author Blue Lake, PhD, who conducted this research as a project scientist in the Department of Bioengineering at UC San Diego, in a press release. “By building an atlas of the different types of cells that make up a healthy kidney, as well as injured and diseased kidneys, we can start to figure out which cell types may be contributing to disease progression. We can get an idea of what changes are happening that cause some injured cell types to repair, and in some cases, transition into a state that can no longer be repaired.”
Despite CKD and acute kidney injury (AKI) being known as uniform diseases, KPMP aims to advance treatment by exploring the high likelihood of them having different points of origin. Gaining familiarity with these different causes and disease pathways can lead to improved treatment.
“We don’t have great treatment options for patients with kidney disease,” said Sanjay Jain, MD, PhD, a Washington University professor of medicine who led this study with five co-corresponding authors, in a press release. “By mapping molecular signatures, we hope to predict which patients are at risk of progressing to kidney failure. This molecular knowledge will, one day, lead us to precise, customized treatments for our patients.”
Similar efforts have also aimed to take new and proactive steps to advance kidney disease treatment.
In January, Trinity Health and Strive Health shared plans to use predictive analytics and machine learning to treat CKD and end-stage kidney disease (ESKD).
Through this collaboration, Strive Health planned to work with Loyola Physician Partners to launch a clinical care model to slow the progression of kidney disease. Using Strive Health technology and services, researchers could gain a better view of the patient experience. Machine learning and predictive analytics also enabled clinicians to take an alternative approach to care, as traditional care methods often focus on the latter stages of the disease, which provide fewer options for solutions.
Another study from May shared how a predictive analytics approach to determine correlations between type 2 diabetes and kidney disease was validated.
The development of the risk prediction model consisted of leveraging clinical data from more than 1,200 patients with type 2 diabetes. The algorithm then uses this data to determine biomarkers for diabetic kidney disease through DNA methylation measurements. DNA methylation is a biological process characterized by small changes in DNA that can alter gene expression.