AI-Driven ECG Analysis Accurately Predicts Right-Side Heart Issues
A deep learning-based electrocardiogram model may enhance clinical evaluations of the heart’s right ventricle, providing a potential alternative to complex imaging.
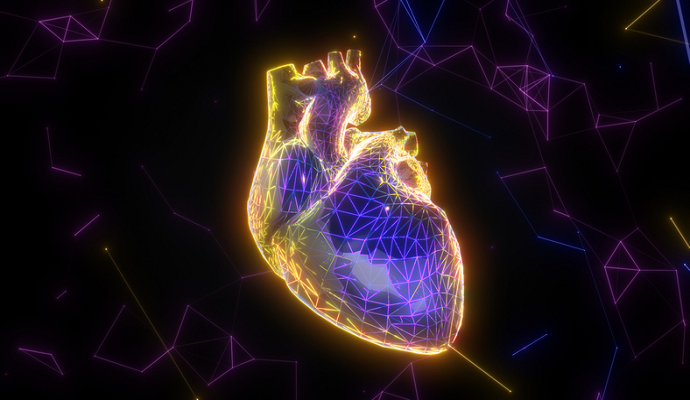
Source: Getty Images
- A research team from the Icahn School of Medicine at Mount Sinai has developed an artificial intelligence (AI) tool to help predict which cardiovascular patients are at increased risk for poor right ventricular function, according to a recent study published in the Journal of the American Heart Association.
One of the primary functions of the heart’s right ventricle is to pump blood to the lungs, but its function can be negatively impacted by cardiovascular disease. To assess right ventricular function, clinicians typically rely on non-invasive medical imaging techniques.
However, the researchers indicated that right ventricular ejection fraction (RVEF) and end‐diastolic volume (RVEDV) are not easily evaluated using traditional methods. They further noted that AI-enabled electrocardiogram (ECG) analysis for right ventricular size and function has been largely unexplored.
To help bridge this gap, the research team trained a deep learning–ECG model to predict right ventricular dilation, dysfunction, and numerical RVEDV and RVEF using data from a 12‐lead ECG paired with reference‐standard cardiac magnetic resonance imaging (MRI) volumetric measurements sourced from patients in the UK Biobank.
The model was then validated at multiple health centers within the Mount Sinai network.
“We aimed to find a better way to assess the health of the heart’s right ventricle, focusing on its ability to pump blood and its size. Traditional methods fall short, which prompted us to explore AI-ECG analysis as a potential solution,” said co-first author Son Q. Duong MD, MS, assistant professor of Pediatrics (Pediatric Cardiology) at Icahn Mount Sinai, in a press release detailing the research. “This novel method could expedite the identification of heart problems, especially in the right ventricle, and potentially lead to earlier and more effective treatment. It holds particular importance for patients with congenital heart disease, who often face issues in the right ventricle.”
The research team assessed the tool based on its accuracy in predicting various heart conditions that impact the right ventricle and its impact on patient survival. The researchers noted that the model’s use of AI helps surface more precise heart information than some of the other tools commonly used in clinical settings.
“This innovative approach departs significantly from traditional methods. Unlike other studies, this research predicts something not easily quantifiable by other common tests, such as the heart ultrasound,” stated co-first author Akhil Vaid, MD, clinical instructor of medicine (Data-Driven and Digital Medicine) at Icahn Mount Sinai.
Despite the tool’s potential, the researchers cautioned that its training relies on existing ECG and MRI data, which may limit its applicability across populations. Further, the model is in the early stages of its development, meaning more work is necessary to assess its applicability and safety.
In the future, the research team plans to continue exploring the tool’s applications in clinical practice by externally validating it in more diverse patient populations and evaluating its usefulness for conditions like cardiomyopathy, congenital heart disease, and pulmonary hypertension.
Deep learning applications in cardiology are being studied more and more as researchers seek to improve screening and risk stratification for heart conditions.
In August, teams from Brigham and Women’s Hospital and Keio University in Japan shared that their recently-developed deep learning model could accurately screen ECGs for signs of atrial septal defects (ASD), a type of congenital heart defect that can damage the cardiovascular system and result in life-threatening complications if not treated in a timely manner.
ASD is traditionally detected by a clinician listening to the heart with a stethoscope or by performing an ECG, but these approaches can be limited and result in defects not being caught until complications arise.
The model, which used ECG and echocardiogram data, was found to be significantly more efficient at flagging ASD than traditional methods.