AI Can Interpret At-Home COVID-19 Tests, Aid Viral Illness Diagnoses
Computerized symptom screenings can help supplement at-home COVID-19 tests and predict whether symptoms are due to COVID-19, influenza, or RSV.
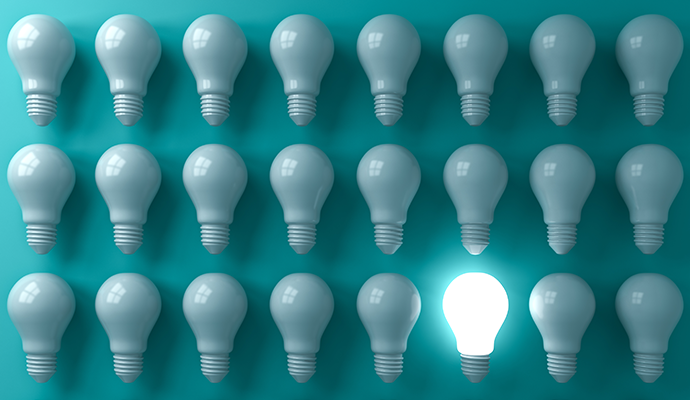
Source: Getty Images
- George Mason University researchers have highlighted how artificial intelligence (AI) can help in the diagnosis of COVID-19 through a series of five papers published in the Journal of Quality Management in Healthcare.
The research explores how the technology can supplement at-home COVID-19 tests and differentiate symptoms caused by the coronavirus, influenza, and respiratory syncytial virus (RSV) for more accurate diagnoses.
The first paper examined the relationship between COVID-19 diagnosis and the timing of symptoms. According to the study, COVID-19 symptoms change over time, with some appearing earlier than others. The researchers sought to investigate whether the order of system occurrence could improve diagnosis before test results are available.
They found that the order of symptom occurrence made a small, but statistically significant, improvement in COVID-19 diagnosis accuracy. They also gained insights into how particular symptoms impacted the test. For example, the research team found that having a runny nose as an early symptom increased the odds of testing positive for COVID, while a runny nose that occurred later decreased the odds.
The second paper looked at the role of symptom clusters in COVID-19 patient triage. Since infection with the virus can manifest in a wide variety of symptoms, the researchers aimed to investigate whether clusters of known neurological, gastrointestinal, and respiratory symptoms could be used to diagnose COVID-19.
READ MORE: Machine-Learning Models Can Help Predict COVID-19 Test Results
The research revealed that symptomatic patients in different age, gender, and race and ethnicity categories presented with different symptom clusters. Thus, COVID-19 cannot be diagnosed from individual symptoms. However, using clusters of symptoms allowed for a more accurate diagnosis.
The third paper investigated the accuracy of at-home COVID-19 tests when combined with computerized symptom screening. At-home rapid antigen tests represent a more convenient, expedited approach to diagnosing COVID-19 compared to other methods, but the accuracy of at-home tests is a challenge, the study noted.
To combat this, the researchers developed an AI-based computerized symptom screening tool designed to predict COVID-19 reverse transcription polymerase chain reaction (RT-PCR) test results. They determined that the use of computerized screening and vaccination status were the most accurate methods to screen patients for COVID-19 infection.
The fourth paper, like the second, also dealt with triaging COVID-19 patients, but focused on how the presentation of multisystemic symptoms could be used to differentiate coronavirus disease from other conditions.
The researchers began by identifying symptoms of COVID-19 reported during the first year of the pandemic. They then surveyed individuals with these symptoms and recent RT-PCR test results to assess the accuracy of diagnosing COVID-19 using those symptoms.
READ MORE: Researchers Assess Federated Learning Models for COVID-19 Diagnostics
They found that certain symptoms, including cough, sore throat, runny nose, dyspnea, and hypoxia, when considered in isolation, were not good predictors of COVID-19 diagnosis. Combinations of particular symptoms, such as fever and cough, were also poor predictors.
However, they found that accuracy was highest when combining symptoms from different body symptoms and lowest when only considering respiratory symptoms. While this method is not accurate every time, and therefore cannot replace testing or other diagnostic measures, the researchers concluded that taking combinations of certain types of symptoms across body systems into consideration can improve patient triage.
The fifth paper outlined the development and validation of an algorithm to predict the probability of COVID-19 infection compared to the flu and flu-like diseases. Differentiating between these conditions based on symptoms is critical during the flu season, and many studies have not taken into account this seasonality and its impact on symptom importance for COVID-19 diagnosis, the study stated.
The researchers determined that differential diagnosis of these diseases relies heavily on demographic characteristics and respiratory, gastrointestinal, and neurological signs and symptoms. The likelihood ratio — a statistical metric used to summarize diagnostic accuracy that describes how likely patients with the disease or outcome are to have a particular result than patients without the disease or outcome — for certain symptoms like cough depended heavily on the flu season. In these cases, the symptom sometimes indicated COVID-19, but other times, it indicated flu or another disease.
However, the study did show that symptoms can be used to screen patients in the community and prior to testing for COVID-19. The researchers recommend that community-based providers follow different signs and symptoms for diagnosing COVID-19 during, and outside of, the flu season.
READ MORE: AI Clinical Decision Support Tool Can Help Predict COVID-19 Prognosis
This is the latest effort to leverage AI and analytics to triage COVID-19 patients.
In February 2021, researchers from the Feinstein Institutes for Medical Research – the science arm of Northwell Health – shared how they designed a predictive analytics model to flag COVID-19 patients at high risk for respiratory failure.
Using data from emergency department visits, such as demographics, labs, and vitals, clinicians and researchers built an algorithm to predict patient risk of respiratory failure within 48 hours. The tool was designed to be integrated into EHRs and clinical workflows to reduce clinician burden during the pandemic. It achieved an average predictive accuracy of 92 percent.
Following the model’s success, Northwell Health revealed plans to implement the solution in a select number of its hospitals.