AI ‘Brain Decoder’ System Translates Human Brain Activity
Researchers have developed an artificial intelligence-based ‘brain decoder’ that can translate brain activity into a continuous stream of text.
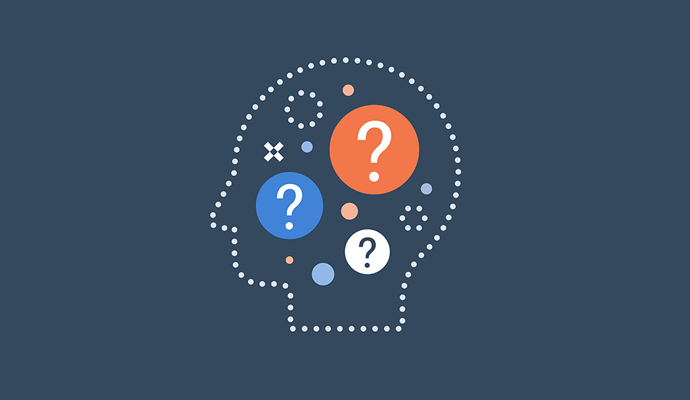
Source: Getty Images
- Researchers from The University of Texas at Austin (UT Austin) have developed an artificial intelligence (AI) system that is capable of translating a person’s brain activity into a continuous stream of text while they are listening to a story or imagining telling a story, according to a study published in Nature Neuroscience this week.
The brain-computer interface, known as a semantic decoder system, has the potential to help people who are mentally conscious, but physically unable to speak, such as stroke victims, communicate again.
A press release published alongside the study indicates that the tool partially relies on a transformer model, similar to those used in Google Bard and ChatGPT. The model also does not require surgical implants, making the approach noninvasive, unlike other systems currently in development.
Instead, the decoder is trained using fMRI brain scan data collected while a participant listens to hours of podcasts. Then, if the participant agrees to have their thoughts decoded, they will be tasked with listening to a new story or imagining themselves telling a story. Using the brain scans generated from this, the system can generate a stream of corresponding text that describes what was being said or thought.
“For a noninvasive method, this is a real leap forward compared to what’s been done before, which is typically single words or short sentences,” explained Alex Huth, PhD, an assistant professor of neuroscience and computer science at UT Austin, in the press release. “We’re getting the model to decode continuous language for extended periods of time with complicated ideas.”
The decoded language that the system outputs is meant to capture the gist of what the participant thought, rather than create a perfect word-for-word transcript. However, about half the time, the decoder can produce text that closely or precisely captures the intended meaning.
Alongside decoding brain activity sourced while patients were listening to stories and podcasts, the model can also decode thoughts related to video-watching. In another experiment, the research team asked study participants to watch four short, silent videos while in the fMRI scanner. The decoder was then able to use these scans to create accurate descriptions of particular events in each video.
Seeking to address some concerns about mental privacy as brain-computer interfaces advance, the researchers also investigated whether successful use of the decoder required participant consent and cooperation.
They found that the system’s outputs were unintelligible and unusable when the tool was used on the brain scans of those on whom the decoder had not been trained and study participants who later resisted to the tool by thinking about other things, like animals or imagining telling their own story.
“We take very seriously the concerns that it could be used for bad purposes and have worked to avoid that,” said Jerry Tang, a doctoral student in computer science at UT Austin. “We want to make sure people only use these types of technologies when they want to and that it helps them.”
When asked about what might happen if future technology advanced to overcome the need for participant consent, Tang indicated that policy and regulation are crucial.
“I think right now, while the technology is in such an early state, it’s important to be proactive by enacting policies that protect people and their privacy,” Tang said. “Regulating what these devices can be used for is also very important.”
For now, use of the tool is not practical outside of a lab setting because of its reliance on an fMRI machine, which participants must spend up to 15 hours lying in for the model to be sufficiently trained.
However, the research team noted that this type of system could transfer to more portable brain-imaging systems, such as functional near-infrared spectroscopy (fNIRS), though the scan resolution would be lower and may present some challenges as a result.
“fNIRS measures where there’s more or less blood flow in the brain at different points in time, which, it turns out, is exactly the same kind of signal that fMRI is measuring,” Huth said. “So, our exact kind of approach should translate to fNIRS.”