AI Algorithm Can Predict Mortality Based on Body Measurements
New research indicated that an AI algorithm could use body composition measurements to predict lung cancer, CVD, and all-cause mortality.
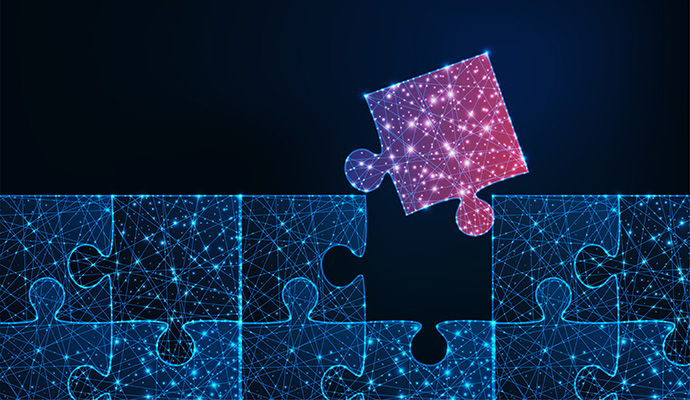
Source: Getty Images
- According to a new study, an artificial intelligence (AI) can assess non-contrast low-dose CT chest scans for cancer screenings and predict outcomes such as lung cancer, cardiovascular disease (CVD), and all-cause mortality.
Although AI continues to grow in healthcare, its application and use in certain areas remains understudied. New research described an AI algorithm that performs body composition assessments of lung cancer screening non-contrast low-dose CT of the chest (LDCT) scans.
In this study, researchers acknowledged that using these measurements in disease risk prediction is understudied. They aimed to determine whether this resource could be useful in foreseeing outcomes such as lung cancer incidence, lung cancer death, CVD death, and all-cause mortality in the National Lung Screening Trial (NLST).
This research process consisted of a secondary analysis of NLST data. From this resource, researchers obtained body composition measurements, such as area and attenuation attributes of skeletal muscle and subcutaneous adipose tissue. These came from baseline LDCT examinations. Researchers used additional proportional hazards models to assess the added value of measurements. Researchers considered this data with and without AI-obtained body composition measurements. Upon analysis, researchers considered other patient characteristics, such as age and medical history.
With a total study population of 20,768, the mean age was 61, and 12,317 were men. Of the full sample, 865 received a lung cancer diagnosis and 4,180 died during follow-up. Researchers found that using AI-obtained body composition measurements informed risk prediction for lung cancer death, as well as all-cause mortality. However, findings indicated lung cancer incidence prediction did not benefit from this process.
The use of AI to predict and define risks related to negative health outcomes is prevalent across the industry.
In April 2022, researchers from the National Institutes of Health Clinical Center created an AI model that used pancreas health and fat level factors to determine type 2 diabetes risk using non-contrast abdominal CT images.
Researchers constructed the algorithm using hundreds of images from various datasets. When analyzing images, they used CT attenuation, pancreatic volume, intrapancreatic fat percentage, and three-dimensional fractal dimension measurements.
They concluded that low pancreas density and high visceral fat correlated with diabetes prevalence. Along with this conclusion, researchers established a level of efficacy associated with this model. The consistent and accurate results produced led researchers to conclude that fully automated analyses of abdominal CT biomarkers are reliable in predicting diabetes.
“This study is a step towards the wider use of automated methods to address clinical challenges,” said study authors Ronald M. Summers, MD, PhD, and Hima Tallam, an MD/PhD student, in a press release. “It may also inform future work investigating the reason for pancreatic changes that occur in patients with diabetes.”
These pieces of research indicate how AI can be used to predict outcomes.