ADA Sessions Showcase Diabetic Retinopathy AI, Artificial Pancreas
This year’s ADA Scientific Sessions highlighted advances in novel devices to enhance diabetes care, including retinopathy risk AI and glucose sensing tech.
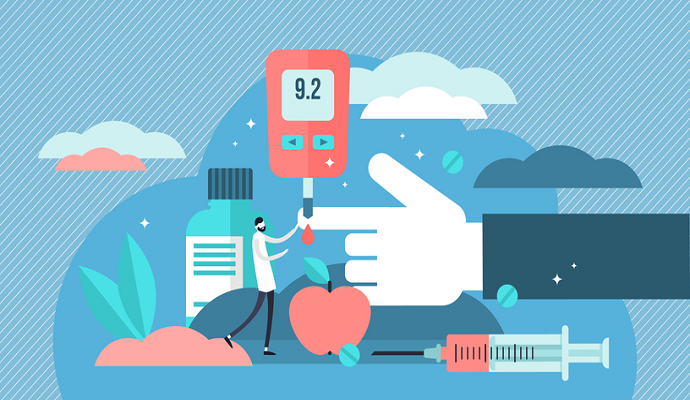
Source: Getty Images
- During the 83rd Scientific Sessions held by the American Diabetes Association (ADA) in San Diego, California, over the weekend, researchers presented studies detailing advancements in diabetes care technology: an artificial intelligence (AI) tool to predict diabetic retinopathy progression and a novel glucose sensing and delivery device for Type 1 diabetes.
These presentations come at a time when AI and other advanced digital health technologies are gaining more momentum in the healthcare industry for various use cases, including chronic disease management.
The first study, ‘Identifying the Risk of Diabetic Retinopathy Progression Using Machine Learning on Ultrawide Field Retinal Images,’ investigated the use of AI to help estimate the risk of diabetic retinopathy progression.
The Centers for Disease Control and Prevention (CDC) estimate that one in three of the 37 million Americans with diabetes will develop the condition, which is the leading cause of blindness in working-age adults in the United States.
Early detection and treatment can help prevent the eye disease’s progression, but for those living with the condition, measuring the risk of progression can be a challenge.
READ MORE: Predictive Analytics Model Forecasts Diabetic Kidney Disease
“Currently, estimating the risk of DR progression is one of the most important, yet difficult tasks for physicians when caring for patients with diabetic eye disease,” said Paolo S. Silva, MD, co-chief of Telemedicine, Beetham Eye Institute, Joslin Diabetes Center and associate professor of Ophthalmology at Harvard Medical School, in the press release. “Our findings show that potentially, the use of machine learning algorithms may further refine the risk of disease progression and personalize screening intervals for patients, possibly reducing costs and improving vision-related outcomes.”
The study demonstrated that by using ultrawide field (UWF) retinal images labeled for baseline diabetic retinopathy severity and progression, validated machine learning (ML) models improved the process of estimating disease progression.
The AI’s predictions for 91 percent of the images were found to either be correct labels or labels with greater estimated progression than the original labels.
The second study, ‘Feasibility of a Prototype Dual Function Glucose Sensing Insulin Delivery Cannula in People with Type 1 Diabetes,’ assessed the viability of an automated insulin delivery (AID) system, or artificial pancreas.
These devices are designed to deliver insulin through pumps based on continuous sensor data about a patient’s glucose levels and are typically used for individuals requiring intensive insulin therapy.
READ MORE: AI Clinical Decision Support Tool Predicts Risk of Diabetes Complications
However, these devices often necessitate multiple devices and separate insertions for the sensor and insulin-delivery cannula. This setup is complex for patients and can lead to additional frustrations that result from asynchronous device replacement timelines.
To address these challenges, the researchers assessed the feasibility of the first single through-the-skin device combining glucose sensing and insulin delivery functions (SynerG).
The device, designed for dual-function glucose sensing-insulin delivery and to synchronize replacement schedules for insulin delivery and glucose measurement functions, was tested in 24 patients. These individuals were diagnosed with Type 1 diabetes and currently on insulin pump therapy (IPT) at the time of the study.
The device was inserted subcutaneously into the abdomen and attached to the pump, and one calibration was performed during the device’s warm-up time. Participants ate a standardized meal 60 minutes post-insertion, and blood glucose levels were measured every ten minutes for 60 minutes before and every 15 minutes for four hours after the meal.
The results showed that insulin was delivered reliably to patients by the device, and the insulin did not interfere with the glucose sensor.
READ MORE: MT Health System Implements Artificial Intelligence Tool for Diabetes Care
“We believe these findings represent a potentially significant step forward in the management of glucose levels for those living with diabetes with the feasibility of a single insertion subcutaneous device able to both sense glucose and infuse insulin. We envision a device like this will help to minimize the burden of care and associated psychological distress for those living with diabetes who require insulin,” said David O'Neal, MD, a professor with the University of Melbourne Department of Medicine and a senior consultant endocrinologist with the Department of Endocrinology at St. Vincent’s Hospital in Australia.
These studies are the latest to evaluate advanced technologies in the context of diabetes care and management.
Last year, researchers at the University of Houston shared that they had developed an AI-based clinical decision support tool to predict the risk of diabetes complications.
The tool, known as Primary Care Forecast, leverages a combination of deep learning (DL) and a patient’s health history to predict which individuals are at higher risk. The solution will be used to enhance the Diabetes Complication Severity Index (DCSI) Progression Tool, which uses patient health history alongside social determinants of health and environmental factors to assess diabetes complication risk. In doing so, the researchers hope to help clinicians become more proactive when treating diabetes and more effectively connect patients with interventions.