$1.2 Million Grant to Support Healthcare Analytics, AI Projects
The Jump ARCHES program has awarded funding to 12 projects focusing on data analytics, machine learning, and predictive modeling to improve healthcare delivery.
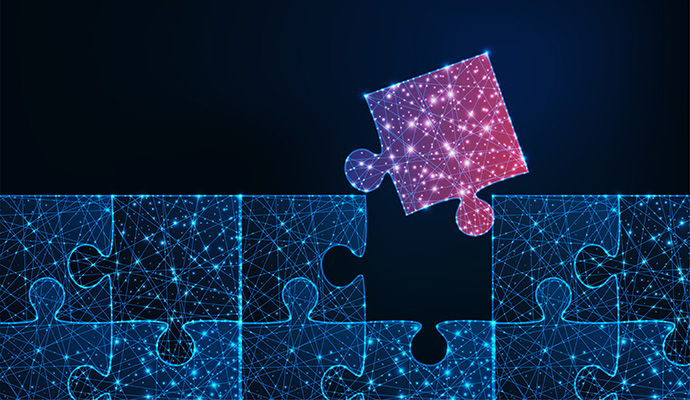
Source: Getty Images
- The Jump Applied Research through Community Health through Engineering and Simulation (ARCHES) research and development program has announced that almost $1.2 million in grant funding has been awarded to 12 projects aiming to improve medical training and healthcare delivery through advanced technologies.
The Jump ARCHES program is a partnership between the University of Illinois Urbana-Champaign (UIUC), the University of Illinois College of Medicine in Peoria (UICOMP), and OSF HealthCare. Funding awarded by the program is used to help clinicians and engineers develop technology and devices to transform healthcare by addressing care barriers such as location, age, and social determinants of health.
The first project, titled ‘STREAM-ED: Simulation to Refine, Enhance and Adapt Management of Emergency,’ seeks to develop models combining event simulation, machine learning (ML), and optimization techniques to tackle emergency department overcrowding and resource utilization.
The next project details a prototype Intelligent Regulatory Change Management (IRCM) system, which would leverage artificial intelligence (AI) and natural language processing (NLP) to help track and assess public policy actions impacting OSF HealthCare.
The press release notes that such a system would allow the health system’s compliance staff to determine appropriate courses of action and identify important changes to improve quality, safety, and privacy risk management.
‘Machine Learning of Standardized DICOM Metadata from Imaging Datasets’ aims to develop an ML-based algorithm capable of categorizing image parameters using signal intensity variations in 2D medical images. This would improve the efficiency of medical image segmentation and enable automated characterization of unknown 3D imaging datasets.
Another project is using imaging segmentation to help create 3D models of neuroblastic tumors for pre-surgical planning at OSF HealthCare Children’s Hospital of Illinois. The project seeks to allow the hospital to shift from 2D imaging to 3D modeling, which would increase the reproducibility of staging analysis and improve models of neuroblastic tumors.
‘Toward Machine-Learned Aortic Arch Measured Diameters’ is tasked with automating the segmentation and clinical measurement of aortic arch diameters from MRI imaging. The project’s early work has shown promising results already, showing a significant correlation between standard clinical measurements and automated ones.
The next proposal seeks to advance brain disease detection and monitoring using deep learning (DL) and multimodal brain imaging data. The project will work to develop ‘brain atlases’ for use in AI-based brain image analysis and computational solutions for automated tumor detection, building on existing research showing that DL improves the identification of brain tumors.
‘Optimizing Pharmacologic Management of Behaviors in Patients with Autism’ will help create an ML model that assists clinicians in choosing appropriate medications and dosages for patients with Autism Spectrum Disorder (ASD). The model will incorporate the patient's genetic information, medical history, and clinician notes to adapt treatment protocols over time.
Another project aims to improve medication adherence for patients with Type 2 diabetes by leveraging predictive analytics to identify individuals at high risk of medication non-adherence, which clinicians can then use to inform strategies to address the underlying causes of non-adherence for their patients.
‘Knowledge Graph Construction with Large Language Models to Predict DKA Occurrence and Severity’ seeks to develop a predictive model that uses named entity recognition- and language modeling-based knowledge graphs to identify high-risk diabetic patients and prevent diabetic ketoacidosis (DKA).
Additional projects include a field experiment evaluating the efficacy of health kiosks supported by community health workers for first-line preventive health screening delivery in underserved areas; an effort to develop a personalized nursing support app to address high nurse turnover; and a proposal to launch community health cafés, which would help provide digital access to healthcare resources to bolster health equity.